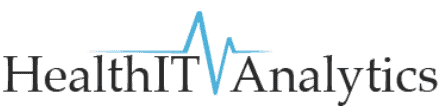
- 11 September, 2018
At Montefiore, Artificial Intelligence Becomes Key to Patient Care
Interest from provider groups is on the rise as machine learning strategies become increasingly sophisticated.
Pilot projects and limited deployments are popping up all over the industry, yet many organizations are tangled in an ongoing struggle to convince skeptical executives to move beyond one-off use cases and embrace AI as an overarching organizational philosophy.
At Montefiore Health System, however, the motto is “full steam ahead.”
For more than three years, Montefiore has been committed to developing a complex, overarching artificial intelligence framework that leverages a massive data lake, cutting-edge infrastructure, and graph databases to inform predictive analytics algorithms targeted to a number of high-value use cases that directly impact patient care.
READ MORE: 5 Steps for Planning a Healthcare Artificial Intelligence Project
The result isn’t just a new screen in the EHR with a risk score and an extra to-do list – it’s a full commitment to a core mission of using all available data to support timely, accurate, proactive decisions across as many clinical realms as possible.
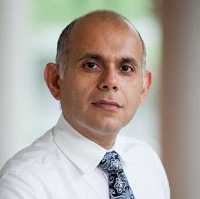
“Our strategy at Montefiore is to build a data-driven and evidence-based health system – essentially a learning healthcare system – that can understand its own population thoroughly, understand and improve its practices, and develop the highest quality of services for the people it serves,” said Parsa Mirhaji, MD, PhD, Director of the Center for Health Data Innovations at Montefiore and the Albert Einstein College of Medicine.
“In order to accomplish that goal, we have created a system that harvests every piece of data that we can possibly find, from our own EMRs and devices to patient-generated data to socioeconomic data from the community. It’s extremely important to use anything we can find that can help us categorize our patients more accurately.”
In 2015, Mirhaji, his colleagues, and a number of industry partners, including Franz Inc. and Intel Corporation, embarked on a project to bring semantic data lake technology to the Bronx-based medical center.
At the time, semantic databases were relatively new to the healthcare industry, and represented an novel way to store large volumes of data and use unique identifiers and natural language processing to perform flexible, adaptable analytics.
READ MORE: Top 4 Basics to Know about Semantic Computing in Healthcare
“The kind of analytics we need to support the future of patient care require the representation of really complex relationships within very different types of data,” said Mirhaji. “Semantic tools are the only ones capable of working with data in that way.”
The first pilot of the semantic data lake had the ambitious goal of identifying all admitted patients at risk of death or intubation within 48 hours.
A few years later, clinicians can now see red flags for admitted patients at elevated risk of mortality three to five days in advance.
Since 2017, providers have also been able to take advantage of advanced sepsis detection tools and a respiratory failure detection algorithm called APPROVE, which can highlight patients at a higher risk of prolonged ventilation up to 48 hours before onset.
“We have achieved a great deal in a short period of time. Our original framework has evolved significantly enough that we have changed the name,” said Mirhaji.
READ MORE: Can Health IT Platforms Support Success as Artificial Intelligence Looms?
“We now call it PALM, the Patient-centered Analytical Learning Machine, and it represents a very new way of interacting with data in healthcare.”
BYPASSING THE MANUAL DATA MANAGEMENT PROCESS
Artificial intelligence tends to require a great deal of data collection, curation, cleaning, and metadata before information can be used to train an algorithm and return a trustworthy result.
In the healthcare industry, where data is notoriously difficult to work with, the process of prepping and feeding data into AI models is a largely manual process – one that consumes significant resources and is often difficult to perform at scale.
“If you talk to any data scientist, they will tell you that 75 percent of their time is spent on data management and data wrangling, and only 15 or 20 percent is devoted to real data science and developing unique analytics,” Mirhaji said.
“With PALM, we have flipped that equation around so that our data scientists can spend 70 percent of their time doing unique analytics. We have spent a lot of time on the underlying architecture so that we can make the most of the creative data science talent we have at our disposal.”
Two unique features of PALM make it possible to take such a hands-off approach to data management, Mirhaji explained.
The first is the way the data lake and its graph repositories are designed around creating on-the-fly relationships between individual elements that can be recombined in nearly infinite ways across disparate data sets.
“We have created data representation and data transformation solutions to more natively translate a wide variety of data into a unified graph that can grow on its own without having a cadre of data analysts, database administrators, and designers to create a data schema and architecture for data integration,” he said.
“The system is capable of building a variety of models on top of the graph representation to transform data and cater to downstream analytics without having to copy the data and move it into data marts or separate databases.”
This core data integration technology allows Montefiore to optimize its data for machine learning and AI without the same investment in man-hours as other, more traditional analytics architecture, Mirhaji added.
“It is a very automated process,” he observed. “There are no human beings deciding how to represent data and deposit it in our graph representations. It’s not entirely schema-less – there are schemas in our data, but they are designed by the algorithm as a result of data input. We have really cut most of the data management processes out of the pipeline.”
LEVERAGING A MEMORABLE INFRASTRUCTURE PARTNERSHIP
The second feature that makes PALM so powerful is the hardware that supports it. Graph technology requires significant computing power, Mirhaji said, and the complexity involved with artificial intelligence demands a new approach to managing memory.
“Distributed graphs can be difficult to scale without an optimized hardware environment, especially if you want to get highly personalized and detailed with your algorithms,” he said. “Creating a truly comprehensive picture of a patient requires bringing together data in a lot of different modalities, and there is an incredible number of data modalities in the clinical space.”
Within the category of “imaging data,” for example, there are at least a dozen variations. In addition to the different images created by x-rays, ultrasound machines, CT scanners, and MRI scanners, there may be high- and low-resolution options, each of which come with their own diagnostic reasoning.
“Each one of these different modalities of images need to be loaded into memory and linked to each other, to their clinical text, radiology notes, lab tests, patient-provided data, and to the biomarkers that surround it,” Mirhaji said.
“That means you’re going to need a lot of random read-write access. There is really no way to write software code to do all of this and manage the parallelism that you need – all of the connectivity, read-write and caching that you need – to solve this problem.”
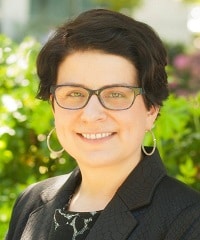
Jennifer Esposito, General Manager of the Health and Life Sciences division of Intel’s Sales and Marketing Group, agrees that the right configuration of system memory and processing power is a core requirement for PALM’s success.
“The databases for something like PALM require a lot of system memory,” she said. “And anything that requires a high degree of memory is going to benefit from a CPU, especially when you compare performance to executing the same problem on a processor that’s built for graphics.”
As part of the constellation of PALM partners, Intel is helping Montefiore tweak its architecture to create the optimal foundation for highly complex tasks.
“We currently have three very different configurations in the works so we can understand what the right combinations are,” said Esposito.
“Leveraging our Xeon processors is important, but we also want to be able to adjust the memory and the storage until we have created a true end-to-end reference architecture that has been optimized to perform in the environment that Montefiore needs.”
The goal is create the smallest possible gap between the processor and the memory, Mirhaji explained.
“By reducing the distance, we can now keep images and caches and metadata available at training time, which makes it much faster, easier, and more scalable to do the type of very complex analytics we are trying to perform,” he said.
INTEGRATING PALM’S ARTIFICIAL INTELLIGENCE INTO THE CLINICAL WORKFLOW
Infrastructure isn’t the only part of PALM that needs to be continually optimized.
The system is designed to create tools that support clinical decision-making at the point of care – and that means they must be introduced into the workflow without causing disruption or frustration for providers.
“I will say right away that I don’t think we have completely solved the problem of integrating analytics seamlessly into the workflow,” Mirhaji acknowledged. “But we are actively working on it, and the clinical workflow has been a very high priority from the beginning of PALM.”
Observing the behavior of clinicians as they interact with Epic, Montefiore’s EHR system, helps Mirhaji and his team refine the way PALM presents information to its end-users.
“We want to improve upon how our practitioners are interacting with the scores and recommendations that the system is generating,” he said.
“We also want to look at how they agree or disagree with the clinical content of the prompt, when they are looking at it, if they are immediately interacting with it or if it takes five different pop-ups to get them to open the dialogue box.”
These interactions are then compared to long-term and short-term patient outcomes, he added.
“We know how many of our patients were flagged by PALM for respiratory failure, and we know how many different practitioners received the notification and how they interacted with it,” he said.
“Did the patient end up being on a vent for an extended period? What happened after they were discharged? Was there a readmission? We follow patients for at least six months after they leave the hospital so we can understand the big picture of how accurate our predictions are and how the alerts and scores are influencing provider decisions.”
Correlating user interaction data with patient outcomes allows Mirhaji’s team to make adjustments to how the information appears to the user and what options are available to surface the data in a meaningful, intuitive manner.
“We are working with our EHR team and our clinical informatics experts to see if visualizations and interactive methods can be used to show analytics to practitioners without producing any form of alert fatigue,” he said.
Ultimately, the goal is for the PALM engine to be more or less invisible to the user. Its insights should be seamlessly integrated into the everyday process of delivering care.
“From the user’s perspective, they are just working with Epic, and that is what we want,” he said. “They don’t get separate training on how to use PALM or how to get alerts and notifications from PALM – it naturally occurs through the same familiar interface that they work with on a daily basis, which reduces the potential for the friction that happens when you tell someone you’re adding something to their system.”
Montefiore will continue to study the impact of artificial intelligence and clinical decision support tools on physician behavior through a three-year grant, Mirhaji said.
“We will be able to use the funding to formally look into the problem and create forums to engage directly with end-users to see how we can augment our design to ensure analytics and AI are integrated well into the workflow,” he said.
SHARING AI BEST PRACTICES WITH THE REST OF THE INDUSTRY
Montefiore is hoping that it is only at the beginning of its journey to create transformational artificial intelligence technologies.
“We now have proof of principle in more ways than one that this is scalable, that it works in very complex settings, and that it’s transferable and generalizable to other domains,” said Mirhaji. “That is very exciting, and we are eager to continue exploring PALM’s potential.”
The health system is starting conversations about how to share its progress with other organizations, both through monetization of the technology and potentially through open-source efforts.
“Currently we are on a path to commercialization, but that doesn’t mean that parts of our solutions and experiences won’t be translated into open source and open API frameworks,” he noted.
“There are certain aspects that will need ongoing support and investment, which will be best suited to a commercial option, but there are also learnings that can be disseminated through open source – it will be a hybrid approach with the goal of maximizing value for adopters.”
Intel, too, aims to share lessons learned with other healthcare organizations looking to harness the potential of AI approaches to predictive analytics and decision-support, added Esposito.
“Montefiore provides a great example of how to focus on specific, well-defined use cases while still planning for the long term,” she said.
“They have built architecture that is flexible enough to enable a huge variety of projects, but have tested their capabilities on a few concrete problems that are already producing measurable benefits.”
Balancing immediate gains with the big picture is essential for making shrewd, financially sound investments in health IT infrastructure, she said.
“Infrastructure isn’t something you buy for a single use case,” she noted. “The realization that you need to invest in agile, future-proof infrastructure might come out of the need to solve a specific problem, but it has to be built in a manner that allows your organization to scale, to innovate, and to grow as technology advances.”
“We can see very clearly from how quickly AI has developed already that infrastructure has to be able to rise to any new challenge an organization is facing,” she continued. “If you don’t have a vision for how to remain flexible and equip your enterprise for change, you might not be making the most of the assets that you’re implementing.”
The ability to creatively and continuously iterate upon a solid infrastructure foundation is a necessity in a rapidly changing artificial intelligence environment, agreed Mirhaji.
“The holy grail of analytics is creating an architecture that doesn’t need to be reinvented every time we want to do something new,” he said. “That requires very smart investment at the beginning to make sure that what we have today will enable us to move into the future without having to stand up custom built solutions that are only good for one project at a time.”
“I think we have demonstrated that it is feasible, and I certainly consider that one of the great achievements of PALM and Montefiore so far.”