To support ubiquitous AI, a Knowledge Graph system will have to fuse and integrate data, not just in representation, but in context (ontologies, metadata, domain knowledge, terminology systems), and time (temporal relationships between components of data). The rich functional and contextual integration of multi-modal, predictive modeling and artificial intelligence is what distinguishes AllegroGraph as a modern, scalable, enterprise analytic platform. AllegroGraph is the first big temporal knowledge graph technology that encapsulates a novel entity-event model natively integrated with domain ontologies and metadata, and dynamic ways of setting the analytics lens on all entities in the system (patient, person, devices, transactions, events, and operations) as prime objects that can be the focus of an analytic (AI, ML, DL) process.
Large enterprises have knowledge repositories that are so big that no amount of vertical scaling will work. When organizations want to conduct new big data analytics, it requires a new effort by the IT department to gather semi-usable data for the data scientists, which can cost millions of dollars, waste valuable time and still not provide a holistic data architecture for querying across all data. ETL, Data Lakes and Property Graphs only exacerbate the problem by creating new data silos. AllegroGraph takes a holistic approach to mixed data, unifying all enterprise data with domain knowledge, including taxonomies, ontologies and industry knowledge – making queries across all data possible, while simplifying and accelerating feature extraction for machine learning.
Enterprise Knowledge Graph Solution Requirements
-
- Deep Integration with LLMs
- Semantic Graph Technology (RDF, OWL, SPARQL, SHAQL, RDF*)
- Scalable Graph Database - Sharding and Federation
- Native Vector Database and Vector Generation
- Native Document Handling and Storage (JSON-LD, JSON)
- Visualization Capabilities
- Natural Language Processing (NLP)
- Taxonomy
- Ontology
- Text Classification
-
- Triple Level Security
- Transactional - ACID
- Temporal Base Reasoning
- Geospatial Features
- Social Networking Algorithm Libraries
- Rules and Logic Programming
- Entity Extractor
- Data Science Integration
- Digital Twin functionality
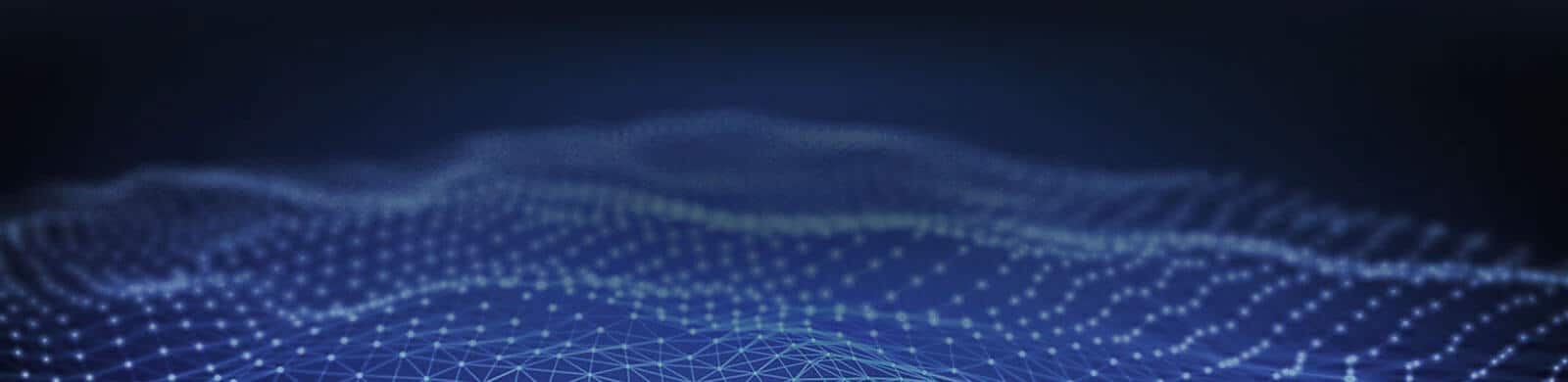
Entity - Event Knowledge Graphs for Data Driven Organizations
- Big Data predictive analytics requires a new data model approach that unifies typical enterprise data with knowledge bases such as taxonomies, ontologies, industry terms and other domain knowledge.
- The Entity-Event Data Model utilized by AllegroGraph puts core ‘entities’ such as customers, patients, students or people of interest at the center and then collects several layers of knowledge related to the entity as ‘events’. Events represent activities that transpire in a temporal context.
- Using this novel data model approach, organizations gain a holistic view of customers, patients, students or important entities and the ability to discover deep connections, uncover new patterns and attain explainable results.
- FedShard™ – Horizontal Scaling that Speeds Complex Queries
Through a patented in-memory federation function, the results from each machine are combined so that the query process appears as if only one database is being accessed, although many different databases and data stores and knowledge bases are actually being accessed and returning results. This unique data federation capability accelerates results for highly complex queries across highly distributed data sets and knowledge bases.
Franz Inc. Your Solution Provider
- AllegroGraph Platform for Scalable Knowledge Graphs
- Technology Innovation Leader
- Graph and AI Industry Expertise
- Training and Support Services
- Consulting and Architecture Professionals
Bloor Research
AllegroGraph and the Franz Inc. Team positioned as a Champion in the Graph Industry