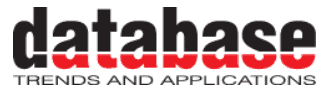
- 31 March, 2016
Database Trends and Applications article – Demystifying Artificial Intelligence
Ever wonder what happened to artificial intelligence? It’s gotten easier and more accessible. In fact, AI’s been embedded into some of the most fundamental aspects of data management, making those critical data-driven processes more celeritous and manageable. Best of all, AI is directly responsible for producing this effect for end users—those who rely on data most to do their jobs.
AI was recently revitalized by advancements in big data and its supporting technologies as part of the cognitive computing movement. Specifically, the current cognitive computing boom is attributable to increased compute power with much greater CPU and memory capabilities such as in-memory processing, as well as reduced costs for infrastructure, software, and storage, and new tools and techniques such as cloud computing, elastic computing, data lakes, and others.
Popular headlines may focus on driverless vehicles, the Internet of Things, and robotics, but the more pragmatic usage of the cognitive computing era involves comparatively simple aspects of integration, analytics, and data management that can now be leveraged for spectacular results.
MACHINE LEARNING AND MORE
“First-order logical reasoning, natural-language processing, speech/voice/vision recognition, neural networks, machine-learning algorithms” are listed by Forrester as chief among AI’s many capabilities, which also include elements of deep learning, smart data, and image recognition. Although these capabilities all depend on semantic technologies, many of their applications are disarmingly easy to use, even for business users.
For instance, machine learning (a subset of predictive analytics in which algorithms determine future uses and action for data based on current and previous ones) is found in a number of different facets of data management. It can enhance data discovery by tailoring results for dashboards and visualizations to suit a specific data type or user preference and do the same for search capabilities. Machine learning algorithms are commonly deployed to expedite the data preparation process and create action based on types of data, such as transformation for business intelligence tools. More sophisticated machine learning applications include recommendation engines, which ecommerce entities can exploit to present offers based on customer behavior, as well as analytics on disparate temporal factors to prevent instances of fraud in the financial space.
INTEGRATION AND NATURAL LANGUAGE PROCESSING
Some of the more cogent deployments of the cognitive computing era combine its myriad capabilities to provide previously unavailable solutions to complicated business problems. Many of these involve integrating unstructured and structured data sources with both historic and real-time data to provide comprehensive analytics almost instantly. Smart data technologies such as RDF graphs and ontologies can enable end users to parse, link, and contextualize disparate data sources of enormous quantities. Machine learning capabilities (including the cognitive algorithms for deep learning and neural networks) initially recognize then predict patterns that greatly accelerate the analytics process and aid in integration efforts by illustrating further context and relationships between data elements.
Natural language processing technologies, which are the basis for sentiment analysis of social media platforms and are deployed in some search engine results, can recognize the intended meanings of terms despite different spellings, diction, connotations, and languages, making integration and analytics efforts more comprehensive. These cognitive computing capabilities are responsible for the parsing of unparalleled quantities of big data in integration and analytics efforts in the healthcare space, facilitating advancements in research and treatment options and testing optimization and enhancing master data management. These capabilities can also incorporate real-time geospatial, weather, news, and industry-specific data to influence marketing, sales, and investment opportunities in any number of verticals. Significantly, natural language processing can also provide explanations for analytics results and recommendations, effectively qualifying quantitative facts.
PRONOUNCED PATTERN RECOGNITION
The profundity of pattern recognition that is fundamental to artificial intelligence and cognitive computing is perhaps most impressive as it relates to speech, image, and visual data. Some of these capabilities are as simple as selecting options from automated phone systems for utilities via voice commands.
They can generate business value by providing analytic insight into videos and pictures on social media platforms, or determining what aspects of marketing and advertising actually resonate with audiences. Some of the more ambitious applications of these technologies include driverless vehicles and robots that perform activities with a limited degree of autonomy based on voice recognition. Nonetheless, simplified and more pervasive versions of these applications include connected cars or smart vehicles, as well as automated virtual assistants that schedule meetings and perform other tasks.
There is a plethora of manifestations of artificial intelligence in use today. Although some of these require significant refinement for widespread, commercial adoption, the core capabilities of cognitive computing (machine learning, NLP, smart data) are influencing almost every facet of data management. More importantly, they are doing so in a way that impacts the work processes of the end user, enabling such users to do their jobs with more celerity, effectively, and thoroughly. In this respect, artificial intelligence is no longer difficult to use.