
- 10 December, 2015
Datanami article – A Semantic Approach to Big Data Governance
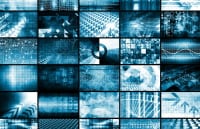
There is a burgeoning need for a standards-based, semantic approach to data governance. It is responsible for worming semantics technologies from the back offices of organizations to the forefront of some of the most pervasive applications of data and their relevance throughout the entire enterprise today.
Big data and its democratization is responsible for demonstrating to organizations the practical value of semantics by enabling them to maintain and improve governance principles over unruly unstructured and semi-structured data. By demonstrating tangible relationships between data elements that discern meaning, standards-based ontological models, vocabularies, and terminology systems are a vital means of implementing big data governance in a sustainable way.
“Governance depends very heavily on preserving meaning,” TopQuadrant co-founder, CMO and VP of Professional Services Robert Coyne reflected. “In these complex ecosystems of systems, data and people, it’s very difficult to preserve meaning and relate meaning through relationships of things that are in different applications and parts of transactions. What semantic standards offer is an operational way to aid the preservation of meaning.”
Common Terms
Semantic technologies play a pivotal role in a key aspect of governance—denoting common terms and definitions that are either used across business units or across the enterprise itself. Vocabularies enhanced by taxonomies can augment those definitions while filling out a repository of such terms by clarifying their meanings and the numerous points of differentiation in spelling and references for a common definition.
According to Franz CEO Jans Aasman: “For internal compliance and compliance with the government, you really want to use the same words for the same thing. Too many times different departments will use different names for the same things. Far worse, they use the same words for different things.”
Most importantly, the utilization of taxonomies (classifications or principles about terms) can account for the inclusion of unstructured and semi-structured data and still provide a unified, organization-wide meaning to disparate references to terms found in sentiment analysis and other forms of big data. This capability is particularly useful for implementing governance policy in programs started after a particular business process or function, which is what frequently occurs “in the real world,” according to Aasman.
Big Data Modeling
Ontological models provide descriptions of data elements and are able to express the relationships between them in a visual manner that is readily discernible to end users. “The idea is to put the human being in a more powerful context where they’re very well plugged in and informed, and have a rich system that they can navigate,” Coyne commented.
In a standards-based environment, these models provide the basis for an accessible way of managing data governance hallmarks such as metadata, reference data, and business glossary mapping. Due to the granular nature of ontologies, their definitions, and the relationships they illuminate (particularly in semantic graph environments), they facilitate an integration and interoperability between various models that would otherwise not be possible—or consume too much time and resources to implement.
Organizations can integrate all of their various forms of metadata (business, technical, security, governance) with ontological models of virtually any type while still ensuring metadata and semantic consistency. According to Aasman: “Data governance is both about the schema and about the data. When you talk about the schema you actually talk more about the ontological thinking, about structuring your data. But the data is more freeform and the data is something that you can capture with vocabularies and terminology systems and taxonomies.”
Meta Relations and More
“Meta relations is just as important as metadata because the meaning is in the relationships between things,” observed Ralph Hodgson, the TopQuadrant co-founder and executive VP and director of TopBraid Technologies.
In addition to facilitating metadata consistency, ontological models also yield information about the meaning of data through their relationships with other data. Meta relations is defined by Hodgson as “the affiliations between things,” and combines with metadata and vocabularies to provide well governed integration of data between sources and uses. This approach is much more applicable to the rich array of options for managing metadata via governance solutions that incorporate semantic technologies, as opposed to those that don’t.
Using a semantic approach, organizations can relate their metadata to specific business functions. There’s also a degree of self-service imbued by utilizing ontological models for basic governance of metadata, reference data, and other data types. End users are able to link different sets of such data, add attributes and metadata about them at will, and update them simultaneously across different systems. “Ontology models, standards-based, are part of the system; they live in the system. They’re run-time models that can be evolved, queried, and extended,” Coyne remarked.
Regulatory Compliance
In addition to the advent of Big Data, one of the key drivers of the contemporary relevance of a semantic approach to big data governance is the onslaught of compliance regulations in numerous industries—particularly finance and health care—in which the governance objectives of “avoiding chaos and reducing risk” noted by Aasman are paramount. Ontologies can directly address compliance issues by creating models based entirely on specific regulations, which can readily integrate with metadata and other facets of compliance based on regulations.
Furthermore, such models can be used to implement a number of critical controls to facilitate compliance. The autonomy granted by the self-service nature of these models is circumscribed by governance solutions that provide sandboxes for immediate trials of changes, prior to an orderly means of implementation according to governance procedures. Additionally, access is both granted and restricted to users according to the roles and responsibilities mandated by governance councils based on instructions directed towards triples, which arguably represent the foundation of semantics. In much the same way, traceability and the provenance of data is also facilitated.
Security
In addition to facilitating internal security via role-based access to data, a standards-based approach to semantics is useful for providing security for external threats as well. When combined with requisite architectures and big data analytics, ontological models can be created to detect the awareness of vulnerabilities. Moreover, organizations can link them to business functions for contingencies. According to Aasman, metadata can also provide insight into security issues.
“You have to look at the metadata of the IP numbers that are trying to invade you,” he said. “What kind of applications are coming at you? What kind of packets? How often is it coming to you? Is this guy related to that guy…The graph database nature of semantic graph databases makes it easier to work with network [security].”
Implementing Big Data Governance
Semantic technologies can greatly abet an organization’s ability to remain consistent in its use of terms and their definitions, metadata, and modeling to achieve goals for regulatory compliance. Moreover, they are essential to determining relationships and ascertaining both context and meaning from them, regardless of different systems. However, they can only augment, and not replace, the foundation of data governance: the roles, responsibilities and rules upon which an enterprise’s data is based.
As Coyne mentioned: “Data governance is growing as a set of practices, and software and systems are an integral part of that. But they’re only a part. What you have at the higher level is communities of practice and policy. Those things are really critical for regulating who is empowered within the organization to make changes.”
About the author: Jelani Harper has written extensively about data management for the past several years. He specializes in semantic technology, big data, and their many different applications.