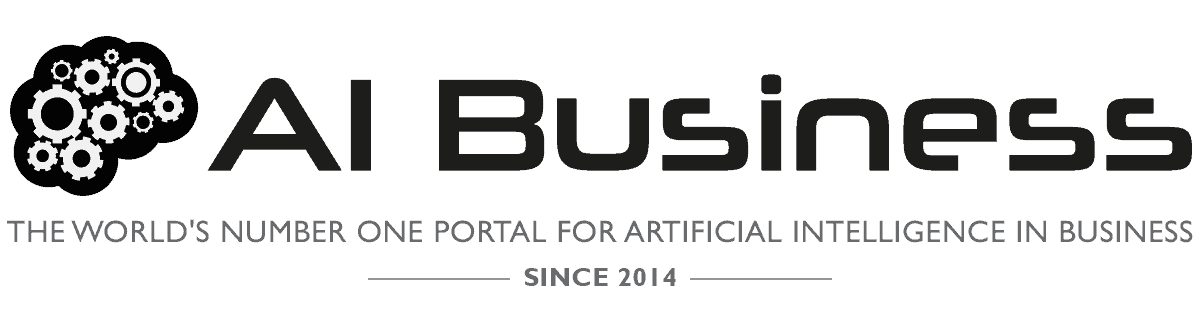
- 28 January, 2020
Deconstructing machine learning: correlation vs. causation, factors, and vectors
Dr. Jans Aasman, CEO, Franz Inc was interviewed for this AI Business article:
Those not quite clear on these concepts run the risk of struggling with explainable AI, and producing models that don’t deliver results successfully impacting business objectives. “Causation is about finding the real factors: the pathways that lead from effect A to B, which can go through effect A1, A2, A3, and A4 before you get to B,” explained Jans Aasman, CEO of graph database developer Franz.
Aasman names a good example of correlation: “It’s a known fact that the rise or decrease in temperature changes murder rates.” In this example, the warmer or colder weather doesn’t necessarily cause more or less killings, but reflects that causative agents for murders have some relationship to the weather.
Once correlation is identified, it’s then necessary to manipulate the inputs and outputs of models to find causation. “If you have a phenomenon and then you do active interference, or an experimentation or something, and then you look at the results, now you can start talking about causation because you either did, or did not do something,” Aasman explained.
Read the full article at AI Business.