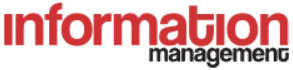
- 11 June, 2015
Info. Mgt. article – Why Data Lakes Require Semantics
Until recently big data was focused on processing massive amounts of simple, flat data. But now, there is a growing need to fuse complex data that comes from both inside and outside of organizations. This evolution is driving the need for new, less expensive and more intelligent analytic frameworks to make better business decisions.
For many years, to support business analytic needs, IT departments invested substantial time and money to pre-process data from various internal data sources into data warehouses and data marts. With the addition of big and complex data this approach is proving to be too slow, too inflexible and with a total cost of ownership (TCO) that has exploded. Additionally, data warehouses struggle to integrate data from outside the enterprise. The warehouse approach is broken for businesses that need better, faster analytics.
Data lakes are relatively new and built largely to help address the TCO of data warehouses and the onslaught of big data. Unlike data warehouses, data lakes use the concept of “pre-processing as little of the data as possible beforehand” to literally toss all the data into the data lake in its native form and fish out what is needed later. Essentially wait to the last possible moment to Extract, Transform, Load (ETL) and integrate the data so called late binding.
But tossing everything into the lake in native formats has a number of challenges that need to be addressed. The late binding approach of data lakes jams the heavy lifting of integrating the data later in the application data use cycle. It saves money upfront, but does nothing to reduce total costs or to solve the key business issue, that being: Make it easier and less costly to get information from data.
According to Nick Heudecker, research director at Gartner, “Data lakes typically begin as ungoverned data stores. Meeting the needs of wider audiences requires curated repositories with governance, semantic consistency and access controls.” Heudecker also says that “ without at least some semblance of information governance, the lake will end up being a collection of disconnected data pools or information silos all in one place.” (Source: Gartner, Gartner Says Beware of Data Lake Fallacy, July 28, 2014)
In order to get value out of data lakes, particularly when complex data is involved, organizations need:
- Semantics for consistent taxonomy
- Meta-data management
- Linking or integration of data otherwise silos stay silos
- Entity level access control/governance
- Curation, provenance and known quality of the data.
Adding Semantic technologies can address many of the issues inherent in Data Lakes if an organization needs to rapidly answer complex, real world questions that require the fusion of data in many dimensions. Semantic Data Lake (SDL) is a semantically integrated, self-descriptive data-repository based on graph (network) representation of multi-source, heterogeneous data, including free text narratives.
Semantic Data Lake Attributes:
- Data linking and integration for internal and external data sources to bring all the data together for better decisions, analytics and answers.
- No schemas to maintain/change. Quicker to adapt to ever-changing business needs.
- Semantic index with control vocabularies and taxonomies to bridge data silos. Accurate results require consistent management of terms and vocabularies.
- Ingest data ONCE and then support any and all new applications, which results in faster time to answers, less expensive to add new data as needs change.
- Secure access control down to individual entities – increasing business compliance and governance require granular control of information access.
- Consistent and flexible meta-data management. Meta-data control enhances the quality of answers and analytics.
- Find non-explicit relationships to gain better understanding, information and answers.
- Complex data model representation for deep analytics, machine learning, AI, Bayesian belief networks.
- Scalability to handle massive data quickly enough to enhance the value of the information.
Healthcare Semantic Data Lake Enabling Precision Medicine
Personalized Medicine is an example where medical professionals need the ability to rapidly answer complex, real world questions that require the fusion of data in many dimensions.
Intel recently showcased a Semantic Data Lake for healthcare at HIMSS 2015, the largest healthcare IT conference in the US. This data lake is built in collaboration between Franz Inc., the Montefiore Medical Center, Intel, Cisco and Cloudera. This Semantic Data Lake will quickly grow to a graph consisting of trillions of edges.
The Healthcare SDL is a massive network of interconnected information that natively and uniformly fuses biomedical terminologies, taxonomy systems, and domain specific knowledge bases. It connects the metadata with the underlying raw and context-independent data from EMRs, medical devices, patient generated data (e.g. Fitbit or personal health devices), patient reported outcomes, genetic and epigenetic tests, publically available environmental, socio-economical and behavioral data, social networks and media, and other non-conventional sources of data, stored at scale and in the cloud.
The SDL integrates, manages, and provides a cost effective platform necessary for organizations to deploy scalable technologies that can enable secondary use of their informational assets.
The SDL provides organizations with a cost effective platform to leverage data to conduct Personalized Medicine as well as: decision support, fraud detection, care management and coordination, quality control, patient safety, clinical errors, liabilities, clinical genetics, etc.), smart applications for mobile and patient engagement, patient centered applications, automated data access and retrieval tools for information sharing and exchange, interactive researcher facing analytic tools, and implementation of self organizing indexing and optimization systems that can constantly and automatically find and repair data inconsistencies in a cloud data center.
Conclusion
Adding Semantics to a data lake can easily transform and integrate unstructured and structured data and query it in real-time, providing critical business intelligence that answers complex questions.