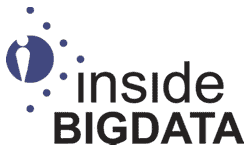
- 22 December, 2015
insideBIGDATA article – Making Big Data More Meaningful through Data Visualization
In this special guest feature, Jans Aasman Ph.D., psychologist and expert in cognitive science as well as CEO of Franz Inc., discusses the use of semantic visual discovery to make big data meaningful. Dr. Aasman was an early innovator in Artificial Intelligence and leading supplier of Semantic Graph Database technology. Dr. Aasman’s previous experience and educational background include: (i) Experimental and cognitive psychology at the University of Groningen, specialization: Psychophysiology, Cognitive Psychology; (ii) Tenured Professor in Industrial Design at the Technical University of Delft. Title of the chair: Informational Ergonomics of Telematics and Intelligent Products; (iii) KPN Research, the research lab of the major Dutch telecommunication company; (iv) Carnegie Mellon University. Visiting Scientist at the Computer Science Department of Prof. Dr. Allan Newell.
We’ve all heard the saying, “a picture says a thousand words.” With today’s millisecond attention spans, communicating a complex topic to any audience – business professional, consumer, doctor, investor, policy-maker, voter — has become more challenging than ever. Some industries are now taking this seriously and investing in new data visualization techniques.
Data visualization is a fundamental part of scientific research. In a scientific journal, pictures certainly do seem to be worth a thousand words, with graphs translating large amounts of data into insightful, visual representations. Cognitive Scientist Moritz Stefaner, says “data visualization is the 21st century’s photojournalism. In a complex world, it makes the invisible visible providing insight into things like algorithmic trading, climate change, tax evasion and government shutdown.”
Data visualization, according to Nathan Yau, a UCLA statistician, is “a representation of data that helps you see what you otherwise would have been blind to if you looked only at the naked source.”
Businesses are increasingly turning to visualization-based data discovery tools, with Gartner estimating a 30 percent compound annual growth rate through 2015. As organizations around the world collect more data than ever, and as Big Data gains popularity as a means of expanding entrepreneurial horizons, visuals can help us understand connections within the data with greater clarity.
What is Data Visualization?
Until recently, spreadsheets have been the main tool used for data analysis and making sense of data. But when you view network data in a spreadsheet it’s difficult to follow a trail – some of the information becomes invisible because it goes two or even 10 levels deep. Using spreadsheets and tables to analyze complex data is too labor intensive and many relationships will remain hidden. When displayed visually, however, data can show relationships that would have otherwise never been clear from a spreadsheet or database.
By visually linking data – relationships become more discoverable.
Discovering New Knowledge through Graph Databases
Graph databases are skyrocketing in popularity and have grown by 500% in the past two years, according to a recent DBMS ranking by DB-Engines. Today there are Semantic Graph databases that use a graph methodology for organizing and linking data. By making these graphs visual, data scientists can navigate the graph and dynamically uncover relationships when two nodes connect.
As opposed to property graphs, which are limited to attributes, Semantic Graphs can be generalized to any depth. And semantic graphs are optimized for both aggregated queries and “pointer-chasing.” With full-query language (SPARQL, W3C) and query optimizers, semantic graph databases are optimized to deal with arbitrary length strings and designed to link disparate data. The semantic graph database paradigm uses ontological systems for typing schema: large, labeled, directed graphs for data; graph pattern matching for query; and recursive query languages for graph analysis. They are especially useful when it comes to highly complex and particularly large data sets.
Data scientists are uncovering new knowledge by linking recorded data with visualization through graph databases, which organize findings for comparison on a graph. With visual representations of many different data points, data scientists can then navigate the graph and dynamically uncover relationships where two nodes (data points) connect. The graph engine, in other words, does the hard work for you.
Predicting your Future Health Status
Healthcare companies and providers are poised to benefit tremendously from uncovering connections within their data. Rather than relying on time-intensive, costly, and limited data marts, medical professionals can analyze and uncover useful connections hidden within huge amounts of data
In one case, semantic graph visualization showed the correlations between peanut allergies and other medical conditions to help improve diagnoses. Here, the visualized relationships show that patients with asthma or dermatitis are more likely to be allergic to peanuts. Without graph visualization, this type of relationship analysis would be difficult and time-consuming, and would also be challenging to represent, due to the many dependent factors that play into the conclusion–in other words, laying out the true cause-and-effect nature of this multi-layered investigation would be like explaining a monarch’s family tree without a pen and paper. That said, when the data is displayed in a graph, the correlations are immediately visible.
This type of data analysis plays a major role in the ongoing evolution of personalized medicine, where medical decisions, practices, and products are tailored to each individual patient, and precision medicine, which takes into account the variability in genes, environment, and lifestyle among patients. With more revealing and meaningful data visualization, medical professionals and scientists can better make correlations to enrich each individual’s treatment.
Uncovering Business Tax Fraud
Another example of using semantic graph visualization can reveal important relationships involves a tax business fraud case. In this investigation, a national tax authority recovered millions of Euros in tax fraud liabilities by using a platform consisting of a Semantic Graph database and an interactive visual navigation tool.
The fraud discovery platform gathered data about companies from public sources, their founders, main executives, and accountants, along with all metadata (addresses, telephone numbers, and emails).
The application also stores information from the vehicle registrations, reverse IP databases, and a comprehensive real estate register. Using an extract-transform-load (ETL) process, this data was modified into Resource Description Framework (RDF) format, and further enriched by several publicly available RDF data sources.
Tax specialists were able to scan and explore the connected data using visual navigation tools for investigative purposes. They were also able to query the data via a visual query generator which allowed them to explore the data without becoming query language experts.
The interactive graph visualization tool provided the tax authority with a new avenue for approaching fraud investigations. Not only could they see the individual actors, but also the network used to set up and redeem the fraudulent notes.
This visualization tool allowed the tax authority to use social network analysis and, more importantly, provided a means of cooperation between various departments and government institutions. In short, the platform allowed analysts to dive deeper into relationships, and to detect the chains within each business that cloaked criminal activity.
Conclusion
Data visualization is critical for taking Big Data and transforming it into meaningful insights. As more industries discover the value of data visualization, organizations that leverage semantic graphs will find themselves well-equipped to map correlations that simple spreadsheets or relational databases would inadvertently hide.