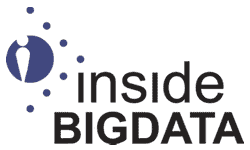
- 12 July, 2016
insideBIGDATA article – The Magic of Visual Querying
A combination of numerous advancements in various technologies—cognitive computing, graph databases, visualizations, and data discovery—is responsible for this phenomenon, which delivers analytics results in a fraction of the time that IT departments typically required for analysis on even proprietary, relational data.The solutions that exploit these developments use interactive visualizations that enable users to look at and select the relevant data for questions, which allows the underlying systems to write the code necessary to perform analytics.
The most competitive solutions provide those results dynamically so users can drag and click between objects to intuitively explore their data and relationships they didn’t even realize existed. The effect is an advanced analytics capability that simplifies query complexity of a magnitude that wasn’t previously possible—and offers astounding results.
The underlying technologies for visual querying of disparate big data sets involve graph databases which are significantly enhanced with semantic technologies. Semantic graphs use ontologies, taxonomies and vocabularies to make it easy to link entities in disparate data sets together using semantic relations and relationship objects. Graph visualizations render those relationships in various ways. Some utilize social network algorithms to determine the shortest path between objects; others use different algorithms to emphasize desired relationships of interest to the user. Virtually all of them do so in a way that allows users to see objects and their symbolic or statistical relationships. The magic is in the way that such visualizations enable querying: codelessly, spontaneously, and between any number of different objects with the simplicity of a click.
Just Asking Questions
Knowledge of SQL or any other querying language is unnecessary for issuing visual queries. In fact, even ‘visual query’ is something of a misnomer since the underlying platforms are responsible for the query-writing process: users are simply asking for connections between entities of interest. Such a procedure is even simpler than typing in questions via the Natural Language Processing capabilities that some cognitive solutions (and more advanced facets of vocabularies and semantic terminology systems) incorporate. Instead, users simply click on objects of interest to determine, for example, the likelihood of a certain medical event relating to another medical event. A query is written to determine that information, but not by the user who can simply click and drag between items of interest to initiate the procedure. This way, users are able to more thoroughly examine their data—structured, unstructured, and semi-structured, regardless of source—and repeatedly ask questions.
Unprecedented Complexity
The demonstrable value of visual querying is based on the way that results of previous questions can impact and shape the selection of additional questions, informing analytic results with more specificity. The visual representation of objects in graph visualizations can, in several instances, determine the most relevant factors to consider when analyzing one’s data. The results of these questions naturally lead themselves to other questions which are based on additional information that users can see—and which might not be apparent with other visualization types.
Some of the more compelling examples of the sort of querying complexity enabled by visual querying is found in the life sciences space, in which researchers can link medical conditions to medications, diagnoses and tests in an almost automatic way. They can do so readily by looking at and clicking on the requisite visual representations, and exchange variables to see, for instance, all of the conditions that contribute to respiratory failure for patients of a certain demographic in a particular locality. Or, they can determine all of the medical billing codes that are relevant to this data. These capabilities enable highly specified, ongoing research in which the most salient factors are identified by the visual representations of graph objects.
Visual Magic
The potency of graph representations is ultimately in the simplicity in which one can issue complicated queries without knowing code. Other visualizations may be able to describe the relationships between objects; browser-based graph visualizations can show them to users, who can render their own descriptions. By simply dragging and clicking on variables, users can readily exchange them with other ones identified as salient by the graph representation. Consequently, they can comb through their data for a discovery-based analytics process that dramatically transforms the value of analytics itself.
Contributed by: Dr. Jans Aasman, Ph.D, CEO of Franz Inc., an early innovator in Artificial Intelligence and leading supplier of Semantic Graph Database technology.