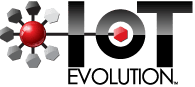
- 16 February, 2017
IoT Evolution article – IoT Will Become Part of Cognitive Computing
Several 2017 predictions have pointed to the increasingly symbiotic relationship between the Internet of Things (IoT) and cognitive computing. The artificial intelligence capabilities of the latter are ideal for the speed and size of the former, while the IoT provides the data quantities needed to optimize the value—and ROI—of cognitive analytics options.
Such predictions, however, are merely indicative of an even larger trend which will solidify the presence of the IoT throughout the datasphere, expand its adoption to reach the rates projected by the end of decade, and simultaneously increase the utility of cognitive computing as a whole. Cognitive platforms will come to routinely encompass data from such a broad spectrum of IoT devices that the IoT will become a de facto component of cognitive computing, becoming practically enveloped by it.
Cognitive computing’s technological acquisition of the IoT will enable the timely aggregation of sensor-driven streamed data with a plethora of sources both internal and external to the enterprise. The application of machine learning, deep learning, neural networks, and other AI algorithms will engender intelligent data to feed into predictive analytics for fully synthesized, time-sensitive cognitive analytic data.
Heightened Analytic Specialization
The use cases enriched by cognitive analytic data stemming from the IoT will range as broadly as the types of data generated in it. Current deployments of the IoT have increasingly impacted supply chain management and route optimization for delivery services, the flourishing smart-device market in the form of domestic devices and connected cars, and healthcare applications for the growing wearables market and ICU sensors. Adoption rates in these verticals are rivaling conventional industrial internet equipment asset management real-time monitoring and predictive maintenance deployments, as well as those in retail and marketing verticals.
IoT’s expansion into cognitive computing in each of these use cases is driven by the unique value that can be inherently gained from cognitive analytic data. This fact is particularly eminent in healthcare deployments of IoT in which AI is required to locate ‘signals’ in massive amounts of unstructured data. Its predictive algorithms are essential for detecting which signals or anomalous data is truly relevant for individual patients
Determining these facts requires the aggregation of IoT data with previous charting history, medical and billing codes, and a variety of other sources including medical journals and clinical trials. Machine learning, and in many cases deep learning, can help to provide points of relevance for patients at an individual level which both inform care and expand treatment options.
Intelligent Iterations
Equally vital to the value produced by including IoT in cognitive computing is the iterative nature it bestows upon predictive analytics as a whole. In addition to providing personalized results for individual use cases, it’s able to refine the results of predictive analytics by incorporating knowledge gained from AI. This iteration is an integral part of the utility which cognitive computing yields, and is manifested in two distinct ways.
First is the iterative nature of AI analytics themselves which take the outcome of current and past results to target the algorithms for future results—creating the same effect on those results in the process. Second is the fact that by combining IoT data with various other data sources and performing some variety of AI analytics, those results can be channeled into additional predictive analytics mechanisms to deliver even more tailored results for a specific use case. This latter iteration represents the full capacity of AI’s inclusion with IoT data because it creates a knowledge base to reuse for additional predictive analytics. Moreover, with each iteration those analytic results become more specific, significantly improving the accuracy of predictions in the process.
Human Tempered Machine Action
The IoT’s impending inclusion into cognitive computing’s AI capabilities is significant for its enhancement of two critical outputs of data-centric processes which have traditionally seemed diametrically opposed to each other: machine-automated action and human-centered decision-making. However, cognitive computing’s absorption of the IoT’s data sources provides the best of each of these capabilities and actually enables such automation to clarify the decision-making process.
Machine learning, deep learning, and neural networks are able to automate data modeling requisites to beget targeted algorithms which improve data management facets of transformation and integration—hastening initial ingestion of data to the aforementioned predictive analytics options. The results of such automation, then, are the foundation for strategic decision-making with a lucidity gleaned from time-sensitive data which is invaluable.
Cognitive Analytic Data
The IoT’s ubiquity is anticipated because of its propensity for creating real-time action from data. Bolstering its applications with cognitive computing’s automation—amalgamating the latter with the former—creates unparalleled predictive analytics accuracy to shape the best of decisions.
About the Author: Dr. Jans Aasman is the CEO of Franz Inc., an early innovator in Artificial Intelligence, Cognitive Computing and global leader in Semantic Graph Database technology. Aasman’s previous experience and educational background include Experimental and cognitive psychology at the University of Groningen, Carnegie Mellon University, Visiting Scientist at the Computer Science Department, Professor in Industrial Design at the Technical University of Delft, Informational Ergonomics of Telematics and Intelligent Products.