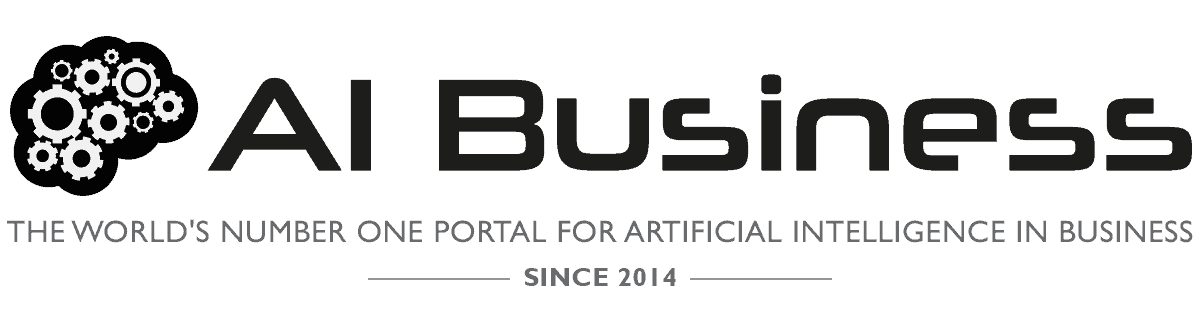
- 1 October, 2018
Is Deep Learning Too Superficial?
Deep learning has been broadly acclaimed for its advanced pattern recognition abilities that are primed for working with data at scale. It can detect non-linear patterns with quantities of variables that make it difficult, if not impossible, for humans to do so. Deep Learning’s strengths are recognizing patterns and translating them into predictions with high accuracy rates.
The Deep Learning Dilemma
Critiques of Deep Learning take issue with how its achievements are produced. Deep Learning needs an inordinate amount of training data to generate reliable results. Moreover, a significant portion of its training data requires annotations for labeled outputs of the model’s objectives. Although there are methods for reducing the data requirements of Deep Learning, (such as transfer learning), there are a number of tasks when such massive amounts of labeled training data just aren’t available.
An even greater limitation is Deep Learning models don’t necessarily understand what they’re analyzing. They can recognize patterns ideal for Natural Language Processing or image recognition systems, but are somewhat restricted in their ability to understand a pattern’s significance and, to a lesser extent, draw inferences from them.
Read the Full Article