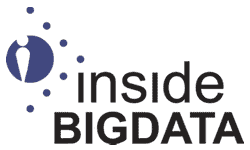
- 3 September, 2020
Little Known Artificial Intelligence Secrets: What Unsupervised Learning Really Means
Dr. Jans Aasman was quoted extensively in this Inside Big Data article:
Unsupervised learning involves training data without labels, in which “the system tries to find kind of a stable set of clusters in your data,” remarked Franz CEO Jans Aasman. “So, the data makes up its own categories.”
This clustering algorithm is partially spatially based and necessitates “kind of dividing all the data in a three-dimensional space into…groups,” Aasman revealed.
Other clustering techniques, however, surmount this limitation and “let the software figure what is the most stable number of clusters that explain the variability of the data the best,” Aasman noted.
“This is how you can start doing precision medicine where you can put people in clusters of things and see if treatments work better for this cluster than that cluster,” Aasman commented.
Factors are input data attributes that impact a machine learning model’s output. PCA not only supports clustering datasets into factors, but also decreasing the number of variables within them so “for each factor you find the most important variable that explains most of the variability,” Aasman indicated.
With machine learning, it’s distinctly possible analytics results are graphs in which “A influences B, B influences C, B also influences E, E inhibits B: [there’s] all these positive, negative, neutral relationships between all these variables,” Aasman explained. “If you want to understand the correlations or what’s in your data then it’s essential to visualize your data.”
Read the full article at Inside Big Data.