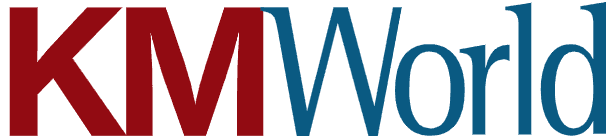
- 8 November, 2019
Monetizing digital asset management: The power of metadata management
Jans Aasman was interviewed for this KMWorld Article:
Advanced analytics: Machine learning delivers a flawless feedback mechanism about which aspects of content resonated with whom, yielding insight into why and how to improve future content. For example, for marketing materials, it would be possible to look at which people were given a document in the past, said Jans Aasman, CEO of Franz. “Then we could do machine learning to learn what was handed out and how effective it was.”
Metadata plays a critical role in machine learning applications for increasing content effectiveness. This technology is largely based on “factors.” Organizations can “take one piece of content and put the official metadata in the factor,” but also put the words used in the document in the factor and other details, such as the customer it was given to and whether there was a sale, Aasman explained. Once these factors are in place, “then the machine learning system will try to figure out what the characteristics are of a factor that leads to a sale,” Aasman said.
Taxonomies: The diversity of content relevant to digital assets, which increasingly includes videos and images alongside documents, requires machine learning-based AI manifestations such as image recognition and computer vision. But, regardless of whether the content involves images or text, the specific words used to describe that content are of the utmost importance for tagging metadata. It is vital to standardize the terms used to describe such metadata (and content) with taxonomies, so organizations have the horizontal means of ascertaining “the type of products people talk about and the type of use cases that they talk about,” Aasman said.
Thus, when analyzing content effectiveness via analytics, users can rely on these hierarchies of business glossary terms for identifiable metrics. Aasman also said that, for DAM, it’s crucial to not only deploy uniform vocabularies for the content’s metadata, but also for the content itself so that “you can derive information from the assets by using NLP [natural language processing] or machine learning or visual recognition.” Metadata may provide the launching point for employing taxonomies for cataloging, but, to be thorough, digital content users must also mine the content itself for the most detailed descriptions of those assets.
Graphing Relationships: Graphing also underpins certain elements of textual or image recognition analytics so that when querying digital assets, organizations can see what Aasman called “multiple properties” across datasets related to one use case—such as the best site for positioning images related to servers, for example.
Read the full article at KMWorld.