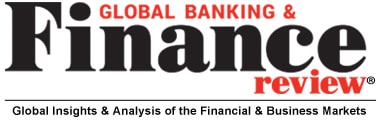
- 12 July, 2018
Optimizing Fraud Management with AI Knowledge Graphs
From Global Banking and Finance Review
This article discusses Knowledge Graphs for Anti-Money Laundering (AML), Suspicious Activity Reports (SAR), counterfeiting and social engineering falsities, as well as synthetic, first-party, and card-not-present fraud.
By compiling fraud-related data into an AI knowledge graph, risk management personnel can also triage those alerts for the right action at the right time. They also get the additive benefit of reusing this graph to decrease other risks for security, loans, or additional financial purposes.
Dr. Aasman goes on to note:
By incorporating AI, these threat maps yields a plethora of information for actually preventing fraud. Supervised learning methods can readily identify what events constitute fraud and which don’t; many of these involve classic machine learning. Unsupervised learning capabilities are influential in determining normal user behavior then pinpointing anomalies contributing to fraud. Perhaps the most effective way AI underpins risk management knowledge graphs is in predicting the likelihood—and when—a specific fraud instance will take place. Once organizations have data for customers, events, and fraud types over a length of time (which could be in as little as a month in the rapidly evolving financial crimes space), they can compute the co-occurrence between events and fraud types.
Read the full article over at Global Banking and Finance Review.