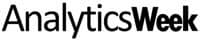
- 16 June, 2022
The Root of the Knowledge Graph Hype: Semantic Technologies
Dr. Jans Aasman, CEO, Franz Inc. was interviewed and quoted for this article:
The mainstream adoption of knowledge graphs is widely acknowledged. More people than ever before know these graph applications are a fundamental part of Artificial Intelligence. The savviest of them realize knowledge graphs typify AI’s reasoning side, as opposed to its statistical foundation (which is exemplified by machine learning).
Far fewer people, however, understand the technological basis for knowledge graphs that makes them sought after by an increasingly broad array of vendors and organizations. If knowledge graphs have finally rose to a position of prominence in the data landscape, it’s almost exclusively due to the semantic graph technologies that make them work to support both machine learning and machine reasoning.
Although there are numerous facets of the semantics that underlie knowledge graphs, the crux of this approach can be summed up in a mere sentence. Or, perhaps, two.
“Semantics is very simple,” posited Franz CEO Jans Aasman. “It just means that you give the same thing in your enterprise the same name, overall in your enterprise.”
That elementary premise becomes the basis for building standardized data models, uniform taxonomies, and vocabularies to represent all data across individual types, schema, formats, and sources. It’s core to the reason knowledge graphs have become so popular, delivering unmatched relationship discernment between data for everything from data preparation to machine reasoning and advanced analytics.
Interconnected Data Assets
The boons of knowledge graphs actually supersede the vast scope of data management essentials, from data engineering to data science. Perhaps their greatest value proposition, which is directly linked to their capability to determine relationships between even seemingly unrelated data, is their extinction of data silos.
“Make sure the same thing has the same name,” Aasman reiterated. “And then, it doesn’t matter the different groups you build and applications. As long as you make sure the person has, always, the same name, the project has always the same name, the product is always the same name, a step in the process always has the same name, the business line always has the same name. Once you do that, all these things automatically integrate and you can share between knowledge graphs.” With this approach data becomes reusable, and the work spent on everything from building data models to building applications becomes reusable as well.
Read the full article at Analytics Week.