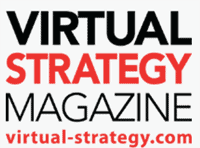
- 6 December, 2016
virtual-strategy.com – Why Semantics & Data Linking is Vital to Artificial Intelligence
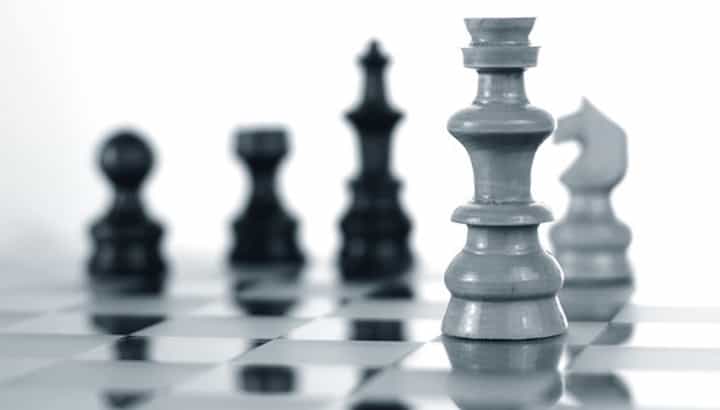
Artificial intelligence and cognitive computing seem to be in the news almost everyday – everything from how AI is now considered a legal driver of a car to Could you fall in love with an AI-enabled robot? Many of these concepts seem far-fetched and some have created concern that making computers too smart could turn computers into criminals and may backfire on the human race someday.
But there have also been great strides to use AI to make precision medicine a reality and predict the outcome of trials by weighing legal evidence and moral questions of right and wrong. The reality is that today many business users, executives and even consumers are already reaping the benefits of natural language processing, machine learning, image recognition and other facets of artificial intelligence, but are blissfully unaware that these phenomena are empowered by semantics and its simple, progressive approach to maximizing data utility.
So how does an AI-enabled robot or autonomous car differ from a typical software application? It all starts with the basic principles of semantic technologies and data linking, which create a basic framework for artificial intelligence and cognitive computing to exist. The linking of data in a declarative, standardized manner is integral to the forms of pattern recognition and machine learning that define cognitive computing.
Data linking and semantics go hand-in-hand, because easily linking data requires a uniform terminology and semantics is key for assigning precise meaning to terms. Therefore, creating a uniform terminology is a good place to start if you want to create an AI application. Once you have a standard vocabulary based on semantics, you can apply data models through a database that can understand and leverage the terminology. This is possible by using a semantic graph database, which also links data and creates self-describing data. By applying machine learning to semantically linked data – you have the beginnings of artificial intelligence.
Let’s start by taking a closer look at Semantics and Data Linking.
Using Semantics to Create a Uniform Terminology
The term semantics denotes meaning and is the branch of linguistics in which precise meaning is applied to words. Semantic technologies implement such explicit, consistent meaning to the terms that are deployed in IT systems. The principal way they do so is with vocabularies and taxonomies. Vocabularies provide meaning to terms; taxonomies facilitate hierarchies of terms for classification purposes. These technologies ensure that the same word is utilized for the same meaning whenever it’s used, which eliminates the commonly found problem in which different departments use different terms for the same thing. Without these technologies there is far greater ambiguity in the meaning of terms, which obfuscates data quality. For example, the term python can either denote a reptile or programming language—semantics regulates which is appropriate.
Semantic Graphs Link Data
Semantic vocabularies become the basis for artificial intelligence and cognitive computing when they are leveraged within Semantic Graph Databases. Graph databases are central to the capability for machines to autonomously ascertain meaning from data. A large part of this capability stems from the way these databases understand and organize data according to semantic statements known as triples—a subject, predicate and object about individual data elements. Triples provide a unique description for each data element in a uniform manner. This standardization allows for different systems to understand data and how they relate to one another, despite differences in structure, data type, or source. Semantic Graph Databases are able to make intelligent inferences about data based on the precedents established by triples, which is one of the core tenets of cognitive computing and artificial intelligence.
The Impact of Semantic Models
Semantic graphs provide the environment in which AI and cognitive systems understand the way that various data elements (such as triples) relate to one another. These facets of semantic technologies are substantially impacted by ontologies—semantic models that are based on the standards-based approach of semantics. When linked together through semantic graph databases, semantic models are able contextualize and specify relationships between data elements at a granular level. Semantic graphs allows semantic models to exist so that all data is inherently linked to one another, which provides the setting for the sharing of data required to discern relationships between them.
Artificial intelligence exploits those relationships in such a way that their underlying applications are able to learn from experience and increase their efficacy over time. Their ability to learn is attributed both to their intelligent inferencing of triples as well as to their inherent understanding of the relationships between data—even those that might be found in seemingly disparate data sets. In fact, the propensity to link data via semantic models on via semantic graphs virtually eliminates the notion of disparate data, since they are related on such a graph via the underlying semantic models, requisite vocabularies and taxonomies.
Self Describing, Smart Data
Semantically tagged data are called ‘smart data’ because they provide a unique description for each data element in a uniform manner. When supported by vocabularies and taxonomies—which provide definitions and hierarchies of definitions to clarify terms and what they mean—those semantic models contextualize and specify relationships between data elements at a fundamental level. A semantic graph allows data models to exist in such a way that all data is inherently linked to one another, which provides the setting for the sharing of data required to discern relationships between them. Artificial intelligence exploits those relationships in such a way that their underlying applications are able to learn from experience and increase their efficacy over time.
Basic Artificial Intelligence: Machine Learning
The foundation for cognitive computing and AI lies in the multitude of facets of semantic technology that provision these applications. The genesis is uniform terminologies underpinned by vocabularies and taxonomies. Semantic Graph databases provide the environment in which semantic statements are used to draw inferences; ontologies enrich the contextualized understanding of data linked together. This confluence is significantly enhanced by machine learning algorithms, the most accessible manifestation of AI that learn from experience and impact future models based on the results of previous ones. The ability to learn over time and operate autonomously is the crux of AI, and depends entirely on semantic technologies.
AI Thrives within Contextual Data Linking
Semantic technologies furnish the environment necessary for the sort of contextualized linking of data upon which artificial intelligence thrives. They help to determine relationships between data at a granular level, which is necessary for the aggregation and integration of the various data sources used in cognitive computing. Furthermore, they provide the foundation for the informed algorithms that are the basis for models that learn over time, adjusting and improving based on the future results of data-driven applications. Their precise descriptions of data, innate ability to contextualize them, and propensity to create models from them greatly exceed those of other technologies.