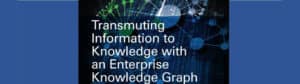
The enterprise knowledge graph for entity 360-views has emerged as one of the most useful graph database technology applications when buttressed by W3C standard semantic technology, modern artificial intelligence, and visual discovery tools. |
Every enterprise has a few core entities that it’s most interested in. For a hospital, this would be the patient; for a telephone company, a customer; and for an intelligence agency, people and organizations of interest. A knowledge graph is a new application of graph technology that collects several layers of knowledge related to an entity of interest.
The Enterprise Knowledge Graph
Not all knowledge graphs are the same; there seem to be three distinct categories:
- Internal operations knowledge graph. Best conceived of as a “company brain,” this knowledge graph focuses on integrating an organization’s assortment of people, skills, experiences, materials, essential company databases, and projects, which greatly improves its self-knowledge and thereby yields competitive advantage. Compiled from combing through myriad databases, including those for human resources, emails, and manifold other sources, this knowledge graph provides the foundation for a rapid, detailed assessment of what knowledge and skills a company has at its disposal—and their relation to one another.
- Intermediary products and services knowledge graph. This graph is designed to create better services and is extremely specific to an organization’s industry, line of business, and area of specialization. For example, Google’s and Yahoo’s search engine endeavors mandate that they collect knowledge about every entity or subject in the world, so they can offer the most relevant, revealing information to their users. LinkedIn’s knowledge graph, on the other hand, details people’s professions, resumes, and career opportunities.1 Again, the relationships between these nodes are paramount.
- External customer knowledge graph. This graph is best thought of as providing as much detail about customers as the first does about the organization itself. Offering the typical 360-degree view of an organization’s clientele, this knowledge graph is dedicated to every point of interaction and piece of information a company can gather about its customers. Typically, the data sources for this graph come from various company silos pertaining to customers; the graph itself highlights the relationships between these pieces of data.
Connecting this Knowledge
The granular customer data from the customer knowledge graph supplies an interesting data source rife for exploitation when analyzed -and acted on- in conjunction with data from the other two graphs. This fact is especially convincing when linking the external customer data with the internal operations data.
Linked Enterprise Data
Linked enterprise data is the nucleus of the aforementioned knowledge graphs. This technology borrows its fundamental concepts from the notion of linked open data. The chief distinction between the two is that the latter involves external, publicly available sources, whereas the former typically revolves around more internal and proprietorial data. Thus, linked open data functions as a precedent of sorts for linked enterprise data.
Universally Identifiable URLs
The fundamental difference between the linked data approach and the more commonly found relational methodology becomes manifest with a cursory comparison between the two. The linked data methodology readily ameliorates these concerns by giving every object in a semantic graph a universally identifiable URL. This URL is the same wherever data is located throughout the enterprise – whether dumped in a data lake or located in a particular repository for a certain purpose.
Deriving Knowledge from Insight
The queryable nature of URLs, which is the crux of the linked enterprise data approach of knowledge graphs, is directly attributable to their ability to transmute insight into established knowledge. The principal way such graphs transform data into proven facts is by taking analytics a step beyond its conventional utility. Most organizations predominantly view the output of analytics as information or “answers” to questions, which are ends in themselves. The highly queryable nature of knowledge graphs, however, surpasses this utility by enabling users to input the results of analytics back into their stacks. In this case, those analytic results are the foundation of concrete knowledge, which is then further used to pinpoint accuracy for additional analytics. Each subsequent wave of analytics only furthers this knowledge, which contributes to the expedient, targeted results provided, for example, by search engines or other applications.
Summary
Overall, enterprise knowledge graphs and their requisite linked enterprise data methodology are swiftly gaining credence throughout the data landscape. The utilitarian nature of these technologies, however, is far too pervasive to be reserved exclusively for the largest, most well-funded organizations in the IT space (such as those identified at the Sunnyvale conference). Small and midsized organizations can significantly enhance their ROI on data-driven technologies by leveraging these graphs to improve their operations, products and services, and overall cognizance of customers. This reality signifies the true utility of the underlying linked data approach at the core of these graphs – they are applicable to any organization and create the same value regardless of the scope or focus of the company deploying them.
![]() Purchase the article from IEEE |
![]() Read the Manuscript |