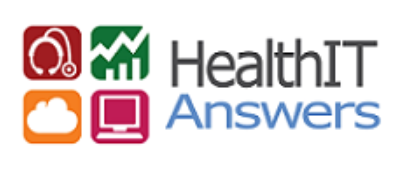
- 17 December, 2024
Fixing Hidden Errors in Medical Systems with NeuroSymbolic AI
By Drs. Jans Aasman and Richard Wallace
This article was published in Health IT Answers.
Imagine querying a medical database to analyze patients with nervous system disorders, only to have the results include unrelated conditions like heart failure and burn injuries. These errors aren’t just technical nuisances—they can have life-or-death consequences in clinical settings.
These errors can stem from the Unified Medical Language System (UMLS), a cornerstone of healthcare data interoperability that unifies over 200 biomedical vocabularies, including SNOMED CT and ICD-10. Despite its critical role, the complexity of UMLS can obscure hidden cycles, potentially leading to inconsistent and inaccurate clinical conclusions.
But by combining the unique strengths of machine learning, logic-based reasoning, and Large Language Models (LLMs), we can clean up UMLS and ensure its massive knowledge graph is accurate, consistent, and usable.
The Importance of UMLS in Biomedical Informatics
UMLS plays a critical role in biomedical informatics by unifying terminologies and mapping relationships across multiple vocabularies, ensuring semantic interoperability. Its Metathesaurus integrates data from various sources, allowing healthcare systems to identify synonymous terms across different medical databases, while the Semantic Network establishes structured relationships between concepts for efficient information retrieval.
Healthcare providers, researchers, and developers rely on UMLS to standardize medical concepts, improve patient care coordination, and enable advanced research. However, inconsistencies in UMLS can lead to incorrect or inefficient results, particularly in systems using symbolic reasoning and hierarchical queries.
The Challenge of Cycles in UMLS
A key issue with the UMLS is the presence of cycles in its relationship structures, particularly in its “narrower” relationships that form the foundation of many hierarchical queries. A cycle occurs when a concept in the UMLS graph is related to itself through a series of narrower or broader relationships. For example, a query designed to retrieve all more specific diagnoses under “nervous system disorder” might mistakenly include unrelated concepts like “heart failure” or “renal dysplasia” due to cycles in the graph.
Read the full article at Health IT Answers.