HealthIT Analytics article – Montefiore Semantic Data Lake Tackles Predictive Analytics
May 31, 2016 – Semantic computing is becoming a hot topic in the healthcare industry as the first wave of big data analytics leaders looks to move beyond the basics of population health management, predictive analytics, and risk stratification.
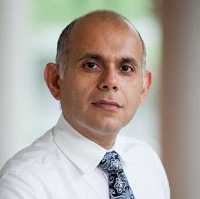
This new approach to analytics eschews the rigid, limited capabilities of the traditional relational database and instead focuses on creating a fluid pool of standardized data elements that can be mixed and matched on the fly to answer a large number of unique queries.
Montefiore Medical Center, in partnership with Franz Inc., is among the first healthcare organizations to invest in a robust semantic data lake as the foundation for advanced clinical decision support and predictive analytics capabilities.
Six months after introducing the concept to readers at HealthITAnalytics.com, Parsa Mirhaji, MD, PhD, has provided an update on Montefiore’s progress with a sophisticated, potentially revolutionary predictive analytics pilot program.
“The Semantic Data Lake is up and running, and it’s doing well,” said Mirhaji, Associate Professor of Systems and Computational Biology and the Director of Clinical Research Informatics at the Albert Einstein College of Medicine and Montefiore Medical Center-Institute for Clinical Translational Research.
READ MORE: At Montefiore, Artificial Intelligence Becomes Key to Patient Care
“Right now, we are still in the middle of a pilot program that uses predictive analytics to flag any patient hospitalized at Montefiore Health System locations, who is at risk of death or of the need for intubation within the following 48 hours, which is the window of opportunity to complete an effective intervention for the course of events.”
As part of a collaboration with the Mayo Clinic, Montefiore is in the process of refining a predictive algorithm founded on retrospective data from more than 68,000 patients across the two institutions. The data lake delivers real-time data for perspective surveillance on real patients, Mirhaji says, using actionable clinical data.
“It creates risk scores based on the patient’s likelihood of a major event within 48 hours,” he explained. “Then there’s another engine that kicks in based on those risk scores and other factors to determine what we can do for that particular patient to avoid the crisis. It can send a personalized checklist of proposed interventions to the practitioner in charge of that case.”
At the moment, the system is still in its pre-clinical validation stage. The algorithm is working in parallel with the traditional care delivery process to test its capabilities, but clinicians are not currently receiving notifications for their patients.
Instead, results are being sent to a group of clinical investigators who are comparing the predictive analytics with real-life patient care procedures to see how well the system is working.
READ MORE: Natural Language Processing, AI to Foster Clinical Decision Tools
“We are very happy with what we’re seeing right now, as the information is very sensitive and very specific,” Mirhaji added. “We can find almost all the high-risk patients in our population with only a one percent error, which is a very good result.”
Read: Top 4 Basics to Know about Semantic Computing in Healthcare
With such impressive early progress, go-live is slated for July of 2016, he said. “At that point, we will start to communicate the notifications directly to providers through our Epic EHR, and we will also collect additional information about whether or not practitioners are actually following the suggestions provided.”
“If they are not adhering to the recommendations, then we will try to get feedback about why, and compare the outcomes of patients being treated according to each decision-making process. That will give us some understanding of the behavioral aspects of using this type of clinical decision support and user acceptance of the way we are communicating these recommendations.”
Because the semantic data lake is a learning system, Mirhaji and his team can feed their results back into the database and treat the information as lessons learned. “All of the scores and predictions that we are collecting will be saved back into the system as data points,” he said. “This will become fodder for future learning.”
READ MORE: Machine Learning 84% Accurate at Flagging Dementia within 2 Years
Montefiore’s semantic computing infrastructure may be able to do much more in the future than flag crisis patients, he added. In conjunction with several clinical partners, the New York-based health system is looking into how the database could aid diabetes management and provide support for patients with sleep disorders, such as apnea.
“Additionally, we are investigating a way to predict behavioral health needs for Montefiore patients to see if there is a relationship between behavioral or mental health issues and outcomes of care,” said Mirhaji.
“That will help us better manage patients with these needs and make improvements to the care delivery system to taking these variabilities into account.”
Medication reconciliation and discharge education are also on the horizon, he said, as well as other use cases involving continuous monitoring and multiple streams of data.
Read: How Machine Learning Could Revolutionize Healthcare Diagnostics
The real-time nature of the semantic predictive analytics architecture is opening up many exciting possibilities, but researchers will need to cultivate a deeper understanding of what specific data elements play a critical role in improving outcomes.
“We must identify the different categories of information we need to deliver to patients to help them understand their regimens,” said Mirhaji. “How can we create very simple and comprehensive instructions about what to do after they leave the hospital?”
“If we can use the semantic data lake to create better characterizations of what is happening with a patient, we can prompt more informed and more impactful clinical decision-making for providers and consistently improve the standard of care for the communities we serve.”