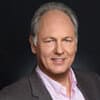
- 1 April, 2018
Navigating Time and Probability in Knowledge Graphs
The market for knowledge graphs is rapidly developing and evolving to solve widely acknowledged deficiencies with data warehouse approaches. Graph databases are providing the foundation for these knowledge graphs and in our enterprise customer base we see two approaches forming: static knowledge graphs and dynamic event driven knowledge graphs.
Static knowledge graphs focus mostly on metadata about entities and the relationships between these entities but they don’t capture ongoing business processes. DBPedia, Geonames and Census or Pubmed are great examples of static knowledge. Dynamic knowledge graphs are used in the enterprise to facilitate internal processes, facilitate the improvement of products or services or gather dynamic knowledge about customers.
Dr. Aasman recently authored an IEEE article describing this evolution of knowledge graphs in the Enterprise and during this presentation we will describe two critical success factors for dynamic knowledge graphs, a uniform way to model, query and interactively navigate time and the power of incorporating probabilities into the graph. The presentation will cover three use cases and live demos showing the confluence of knowledge via machine learning, visual querying, distributed graph databases, and big data not only displays links between objects, but also quantifies the probability of their occurrence.
View a recording of the webcast on our YouTube channel.
Slides are available for download.