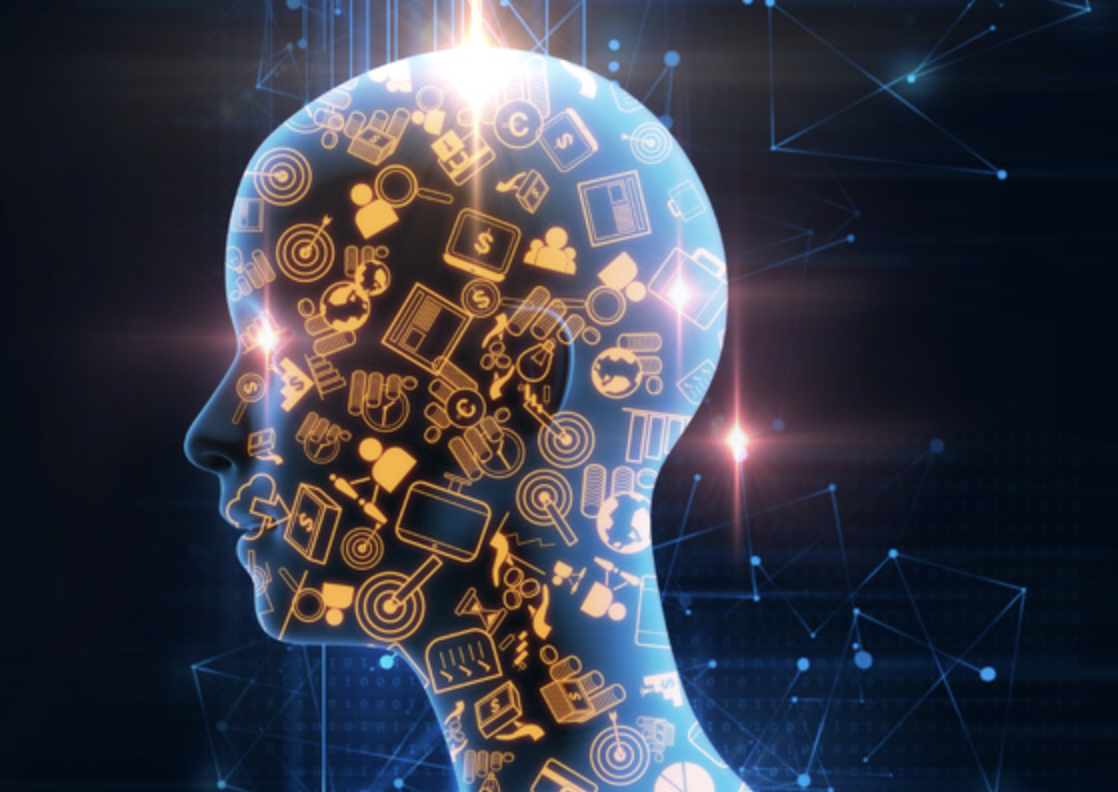
- 17 April, 2024
Can Neuro-Symbolic AI Solve AI’s Weaknesses?
Dr. Jans Aasman was interviewed about Neuro-symbolic AI by TDWI.
Neuro-symbolic AI integrates several technologies to let enterprises efficiently solve complex problems and queries demanding reasoning skills despite having limited data. Dr. Jans Aasman, CEO of Franz, Inc., explains the benefits, downsides, and use cases of neuro-symbolic AI as well as how to know it’s time to consider the technology for your enterprise.
Upside: What is Neuro-symbolic AI?
Dr. Jans Aasman: Neuro-symbolic AI is an advanced approach that integrates machine learning, neural network decision-making, symbolic logic and reasoning, and the capabilities of large language models. This combination enables the ability to efficiently solve complex problems and queries demanding reasoning skills while facilitating effective learning with limited data.
By combining these approaches, the AI facilitates secondary reasoning, allowing for more nuanced inferences. This secondary reasoning not only leads to superior decision-making but also generates decisions that are understandable and explainable to humans, marking a substantial advancement in the field of artificial intelligence.
In what ways does neuro-symbolic AI extend or build upon existing AI methodologies?
Neuro-symbolic AI is designed to capitalize on the strengths of each approach to overcome their respective weaknesses, leading to AI systems that can both reason with human-like logic and adapt to new situations through learning. The tangible objective is to enhance trust in AI systems by improving reasoning, classification, prediction, and contextual understanding.
What benefits does neuro-symbolic AI offer?
The features of Neuro-symbolic AI address the limitations of purely neural network-based or purely symbolic AI systems. Here are the main ones that come to mind:
- Enhanced reasoning and generalization. Neuro-symbolic AI can reason over knowledge represented symbolically, allowing it to generalize from fewer examples than neural networks, which often require large data sets to learn.
- Improved interpretability. Symbolic components allow the AI to explain its decisions and reasoning processes in a human-understandable way, addressing the “black box” issue commonly associated with deep learning models.
- Flexibility in data requirements. This approach can work with both big and small data. Symbolic AI can handle tasks with limited data through predefined rules and logic; neural networks can process and learn from large data sets.
- Error handling. Symbolic systems can be more resistant to errors because they follow explicit rules. Combining this with neural networks can create systems that are both adaptable and precise.
- Learning efficiency. Neuro-symbolic systems can potentially learn more efficiently, combining the rapid pattern recognition of neural networks with the structured knowledge representation of symbolic AI.
- Domain knowledge integration. The ability to integrate expert domain knowledge in a structured form, such as ontologies and knowledge graphs, allows these systems to make informed decisions in specific areas.
- Combination of learning and reasoning. These systems can both learn from data (just like neural networks) and reason about data (like symbolic systems do), making them versatile for a wide range of applications.
Read the full article at TDWI’s Upside.