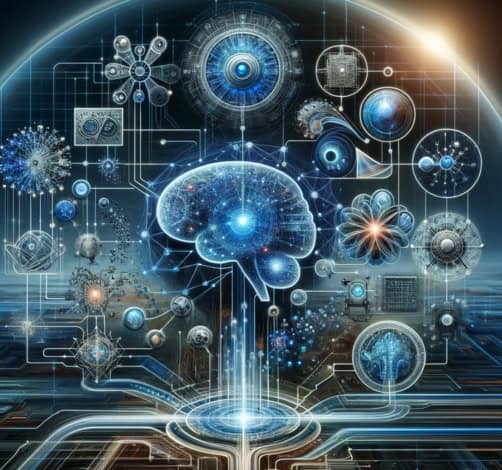
- 23 May, 2024
How a Neuro-Symbolic AI Approach Can Improve Trust in AI Apps
As a cognitive scientist, I’ve been immersed in AI for more than 30 years – specifically in speech and natural language understanding, as well as machine-based learning and rule-based decision-making. Progress in our field is always uneven, unfolding in fits and starts. Those of us in the AI field have witnessed multiple “AI winters” over the decades, yet we continue to advance the vision of AI. With the emergence of ChatGPT and other generative AI large language models (LLMs), we have reached a tipping point in the trajectory of AI – a juncture I never thought we’d achieve in my lifetime.
But LLMs on their own are only one piece of the AI puzzle. The real leap forward for AI comes with combining the different approaches of AI into a single system that utilizes the unique strengths of each approach, while simultaneously addressing their inherent weaknesses.
By integrating machine learning (statistical AI), neural network-based decision-making (neuro AI), symbolic logic and reasoning (symbolic AI), and the powerful capabilities of large language models (generative AI), we can solve complex problems that require reasoning abilities, while also learning efficiently with limited data and expanding the applicability of AI across a broader array of tasks. Importantly, the blending of symbolic AI, statistical AI, and neuro AI with generative AI produces decisions that are understandable to humans and explainable, an important step in the progression of AI.
The synergy of these technologies is of particular interest to enterprises because it offers the potential to significantly enhance trust in AI inferences. By fostering more transparent and explainable AI systems, organizations can achieve a higher level of confidence in the decisions and insights generated by their AI systems, paving the way for more reliable and understandable AI-driven solutions.
The Role of Knowledge Graphs
Semantic knowledge graphs are fundamental to neuro-symbolic AI. The first generation of knowledge graphs, emerging around 15 years ago, primarily utilized symbolic logic and rule-based approaches to generate valuable insights. While systems founded on logic or rules are known for their reliability and consistency, they frequently face challenges with complexity and ongoing maintenance.
The second generation, beginning approximately 10 years ago, incorporated classical machine learning and graph neural networks to draw inferences directly from the knowledge graph data. This innovation introduced abilities to classify objects, predict connections within the knowledge graph, or forecast events concerning customers, aircraft, or patients. These machine learning techniques are amazing at uncovering new rules and patterns from extensive datasets, but they unfortunately also often suffer from opacity and ingrained biases.
The latest, third generation of knowledge graphs, which started around two years ago, integrates the capabilities of large language models (LLMs) and local vector stores (or retrieval-augmented generation – RAG) into the knowledge graph framework. The introduction of LLMs has revolutionized our ability to make inferences about entities within the knowledge graph, leveraging the immense computational power of LLMs. This generation has significantly simplified the creation of ontologies, taxonomies, and the formulation of queries and rules. However, LLMs introduce their own challenges, notably a skepticism towards the reliability of their inferences, necessitating rigorous verification of each inference made.
Let’s explore some ways in which AI systems can complement each other, keeping in mind that these examples represent just a small portion of the potential applications.
Read the full article at Dataversity.