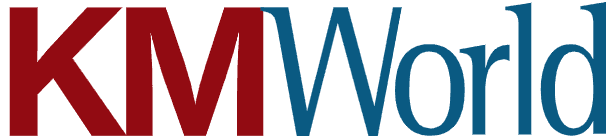
- 9 March, 2021
Understanding What Matters With Text Analytics and NLP
Dr. Jans Aasman was quoted extensively in this KMWorld Article:
Whether employing traditional rulesbased approaches to text analytics or leveraging more modern machine learning strategies, users must initially train the systems on relevant business domains. One way to do so is with comprehensive taxonomies of terms, their synonyms, and their meanings—which are traditionally associated with rules-based models. According to Franz CEO Jans Aasman, “There’s a part of NLP where people create taxonomies and ontologies. That is just a very acceptable way of doing NLP.” Historically, such defined hierarchies of vocabularies were paired with rules to find patterns in text and create actions such as classifications or entity extraction.
The trade-off between this approach and the taxonomic one is clear: Organizations can forsake the extensive time required to build taxonomies by simply using annotated training data. The objective is to “just throw statistics and machine learning at the problem so it will all automatically work,” Aasman said. Although reduced time-to-value is an advantage of the deep learning approach, there are issues to consider, including the following:
♦ Training data: Machine learning models require immense amounts of training data, which organizations might not have for their domains. Transfer learning solves this problem by enabling subject matter experts to upload a couple of hundred examples (instead of thousands), highlight them, and teach dynamic models “the representative entities, key-value pairs, and classes they’re trying to derive from these documents,” Wilde noted.
♦ Controlled vocabularies: Transformers and techniques such as Bidirectional Encoder Representations from Transformers (BERT) reduce the training data quantities for machine learning models, broaden the array of training data that’s relevant, and implement a controlled vocabulary that otherwise isn’t as defined as taxonomic ones. Thus, organizations can take a phrase and “generate a similar phrase that means the same, but can be used in multiple reports in a controlled way,” Mishra said. Additionally, it’s possible to simply purchase libraries of terms and definitions. “Many companies end
up buying those things to be able to incorporate those capabilities,” Shankar added.
♦ Practical knowledge: Exclusively using machine learning models to train text analytics decreases the real-world understanding and applicability of text. “People that do machine learning don’t want to spend the effort to create a vocabulary or the pragmatics or the semantics,” Aasman noted. “Machine learning has a place in all of this, but it misses part of the whole future solution where we have systems that understand what people are talking about.”
Read the full article at KMWorld.