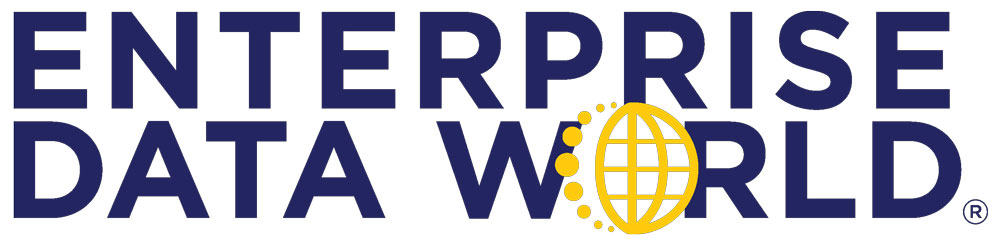
- 30 November, 2023
Using Knowledge Graphs and LLMs for Deep Entity Exploration – EDW 2024
Using Knowledge Graphs and LLMs for Deep Entity Exploration
Wednesday, March 27, 2024
Gartner and Forrester emphasize the importance of constructing knowledge graphs to connect data silos. By doing so, companies can achieve a comprehensive enterprise data fabric solution that enables deeper analytics but also optimizes AI investments. Usually, too much emphasis is placed on structured data but the reality is that in many enterprises there is even more information and knowledge hidden in unstructured data. So the ultimate goal is to provide non-technical end users with the additional ability to query across the business knowledge contained in unstructured data, business correspondence, financial files, and contracts.
Recent advancements, like LLMs and vector-enabled knowledge graphs, permit a blend of natural language and structured queries to retrieve data from documents putting the goal one step closer to delivering queries that span your data fabric.
During our demonstration, we will compare and contrast three approaches data architects should consider in developing a knowledge graph-based approach to connecting valuable enterprise and industry data. For demonstration purposes we will use a collection of legal documents that pertain to the financial industry, in our case we take the entire collection of FINRA rules that are publicly available on the web.
1. Standard LLM Interaction: We demonstrate best practices for querying a legal contract via an LLM website. Are the answers sufficient and do they provide the depth and references necessary for users? Could a better answer have been in another document?
2. LLM combined with web search: Combining web search with an LLM greatly improves answer quality for more complex questions and in some cases, rule references are provided. But are the references specific enough to point back into our local documents?
3, Contract Knowledge Graph as the Source of Truth for LLMs: By storing the example FINRA contracts along with vector embeddings in a knowledge graph, we yield accurate answers directly linked to specific rule passages in the documents that provide evidence for the answers. In addition, we show the deep entity connections exposed in the graph as a result of all the cross references.
This presentation will show users how to efficiently link siloed knowledge and query across documents with natural language techniques for richer insights on entities of interest.