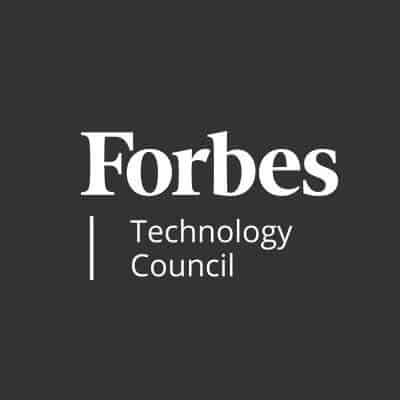
- 23 May, 2019
Creating Explainable AI With Rules
Franz’s CEO, Jans Aasman’s recent Forbes article:
There’s a fascinating dichotomy in artificial intelligence between statistics and rules, machine learning and expert systems. Newcomers to artificial intelligence (AI) regard machine learning as innately superior to brittle rules-based systems, while the history of this field reveals both rules and probabilistic learning are integral components of AI.
This fact is perhaps nowhere truer than in establishing explainable AI, which is central to the long-term business value of AI front-office use cases.
Granted, simple machine learning can automate backend processes. However, the full extent of deep learning or complex neural networks — which are much more accurate than basic machine learning — for mission-critical decision-making and action requires explainability.
Using rules (and rules-based systems) to explicate machine learning results creates explainable AI. Many of the far-reaching applications of AI at the enterprise level — deploying it to combat financial crimes, to predict an individual’s immediate and long-term future in health care, for example — require explainable AI that’s fair, transparent and regulatory compliant.
Rules can explain machine learning results for these purposes and others.
Read the full article at Forbes