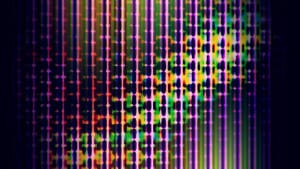
- 25 April, 2020
Natural Language Processing and Machine Learning in AllegroGraph
The majority of our customers build Knowledge Graphs with Natural Language and Machine learning components. Because of this trend AllegroGraph now offers strong support for the use of Natural Language Processing and Machine learning.
Franz Inc has a team of NLP engineers and Taxonomy experts that can help with building turn-key solutions. In general however, our customers already have some expertise in house. In those cases we train customers in how to take the output of NLP and ML processing and turn that into an efficient Knowledge Graph based on best practices in the industry.
This document primarily describes the NLP and ML plug-in AllegroGraph.
Note that many enterprises already have a data science team with NLP experts that use modern open source NLP tools like Spacy, Gensim or Polyglot, or Machine Learning based NLP tools like BERT and Scikit-Learn. In another blog about Document Handling we describe a pipeline of how to deal with NLP in Document Knowledge Graphs by using our NLP and ML plugin and mix that with open source tools.
PlugIn features for Natural Language Processing and Machine Learning in AllegroGraph.
Here is the outline of the plugin features that we are going to describe in more detail.
Machine learning
- data acquisition
- classifier training
- feature extraction support
- performance analysis
- model persistence
NLP
- handling languages
- handling dictionaries
- tokenization
- entity extraction
- Sentiment analysis
- basic pattern matching
SPARQL Access
- Future development
Machine Learning
ML: Data Acquisition
Given that the NLP and ML functions operate within AllegroGraph, after loading the plugins, data acquisition can be performed directly from the triple-store, which drastically simplifies the data scientist workflow. However, if the data is not in AllegroGraph yet we can also import it directly from ten formats of triples or we can use our additional capabilities to import from CSV/JSON/JSON-LD.
Part of the Data Acquisition is also that we need to pre-process the data for training so we provide these three functions:
- prepare-training-data
- split-dev-test
- equalize (for resampling)
Machine Learning: Classifiers
- Currently we provide simple linear classifiers. In case there’s a need for neural net or other advanced classifiers, those can be integrated on-demand.
- We also provide support for online learning (online machine learning is an ML method in which data becomes available in a sequential order and is used to update the best predictor for future data at each step, as opposed to batch learning techniques which generate the best predictor by learning on the entire training data set at once). This feature is useful for many real-world data sets that are constantly updated.
- The default classifiers available are Averaged Perceptron and AROW
Machine Learning: Feature Extraction
Each classifier is expecting a vector of features: either feature indices (indicative features) or pairs of numbers (index – value). These are obtained in a two-step process:
1. A classifier-specific extract-features method should be defined that will return raw feature vector with features identified by strings of the following form: prefix|feature.
The prefix should be provided as a keyword argument to the collect-features method call, and it is used to distinguish similar features from different sources (for instance, for distinct predicates).
2. Those features will be automatically transformed to unique integer ids. The resulting feature vector of indicator features may look like the following: #(1 123 2999 …)
Note that these features may be persisted to AllegroGraph for repeated re-use (e.g. for experimenting with classifier hyperparameter tuning or different classification models).
Many possible features may be extracted from data, but there is a set of common ones, such as:
1. individual tokens of the text field
2. ngrams (of a specified order) of the text field
3. presence of a token in a specific dictionary (like, the dictionary of slang words)
4. presence/value of a certain predicate for the subject of the current triple
5. length of the text
And in case the user has a need for special types of tokens we can write specific token methods, here is an example (in Lisp) that produces an indicator feature of a presence of emojis in the text:
(defmethod collect-features ((method (eql :emoji)) toks &key pred)
(dolist (tok toks)
(when (some #'(lambda (code)
(or (<= #x1F600 code #x1F64F)
(<= #x1F650 code #x1F67F)
(<= #x1F680 code #x1F6FF)))
(map 'vector #'char-code tok))
(return (list "emoji")))))
Machine Learning: Integration with Spacy
The NLP and ML community invents new features and capabilities at an incredible speed. Way faster than any database company can keep up with. So why not embrace that? Whenever we need something that we don’t have in AllegroGraph yet we can call out to Spacy or any other external NLP tool. Here is an example of using feature extraction from Spacy to collect indicator features of the text dependency parse relations:
(defmethod collect-features ((method (eql :dep)) deps &key pred dep-type dep-labels)
(loop :for ds :in deps :nconc
(loop :for dep :in ds
:when (and (member (dep-tag dep) dep-labels)
(dep-head dep)
(dep-tok dep))
:collect (format nil "dep|~a|~a_~a"
dep-type
(tok-word (dep-head dep)
(tok-word (dep-tok dep))))))
The demonstrated integration uses Spacy Docker instance and its HTTP API.
Machine Learning: Classifier Analysis
We provide all the basic tools and metrics for classifier quality analysis:
- accuracy
- f1, precision, recall
- confusion matrix
- and an aggregated classification report
Machine Learning: Model Persistence
The idea behind model persistence is that all the data can be stored in AllegroGraph, including features and classifier models. AllegroGraph stores classifiers directly as triples. This is a far more robust and language-independent approach than currently popular among data scientists reliance on Python pickle files. For the storage we provide a basic triple-based format, so it is also possible to interchange the models using standard RDF data formats.
The biggest advantage of this approach is that when adding text to AllegroGraph we don’t have to move the data externally to perform the classification but can keep the whole pipeline entirely internal.
Natural Language Procession (NLP)
NLP: Language Packs
Most of the NLP tools are language-dependent: i.e. there’s a general function that uses language-specific model/rules/etc. In AllegroGraph, support for particular languages is provided on-demand and all the language-specific is grouped in the so called “language pack” or langpack, for short – a directory with a number of text and binary files with predefined names.
Currently, the langpack for English is provided at nlp/langs/en.zip, with the following files:
- contractions.txt – a dictionary of contractions
- abbrs.txt – a dictionary of abbreviations
- stopwords.txt – a dictionary of stopwords
- pos-dict.txt – positive sentiment words
- neg-dict.txt – negative sentiment words
- word-tok.txt – a list of word tokenization rules
Additionally, we use a general dictionary, a word-form dictionary (obtained from Wiktionary), and custom lexicons.
Loading a langpack for a particular language is performed using load-langpack.
Creating a langpack is just a matter of adding the properly named files to the directory and can be done manually. The names of the files should correspond to the names of the dictionary variables that will be filled by the pack. The dictionaries that don’t have a corresponding file will be just skipped.We have just finished creating a langpack for Spanish and it will be published soon. In case you need other dictionaries we use our AG/Spacy infrastructure. Spacy recently added a comprehensive list of new languages:
NLP: Dictionaries
Dictionaries are read from the language packs or other sources and are kept in memory as language-specific hash-tables. Alongside support for storing the dictionaries as text files, there are also utilities for working with them as triples and putting them into the triple store.
Note that we at Franz Inc specialize in Taxonomy Building using various commercial taxonomy building tools. All these tools can now export these taxonomies as a mix of SKOS taxonomies and OWL. We have several functions to read directly from these SKOS taxonomies and turn them into dictionaries that support efficient phrase-level lookup.
NLP: Tokenization
Tokenization is performed using a time-proven rule-based approach. There are 3 levels of tokenization that have both a corresponding specific utility function and an :output format of the tokenize function:
:parags – splits the text into a list of lists of tokens for paragraphs and sentences in each paragraph
:sents – splits the text into a list of tokens for each sentence
:words – splits the text into a plain list of tokens
Paragraph-level tokenization considers newlines as paragraph delimiters. Sentence-level tokenization is geared towards western-style writing that uses dot and other punctuation marks to delimit sentences. It is, currently, hard-coded, but if the need arises, additional handling may be added for other writing systems. Word-level tokenization is performed using a language-specific set of rules.
NLP: Entity Extraction
Entity extraction is performed by efficient matching (exactly or fuzzy) of the token sequences to the existing dictionary structure.
It is expected that the entities come from the triple store and there’s a special utility function that builds lookup dictionaries from all the triples of the repository identified by certain graphs that have a skos:prefLabel or skos:altLabel property. The lookup may be case-insensitive with the exception of abbreviations (default) or case-sensitive.
Similar to entity extraction, there’s also support for spotting sentiment words. It is performed using the positive/negative words dictionaries from the langpack.
One feature that we needed to develop for our customers is ‘heuristic entity extraction’ . In case you want to extract complicated product names from text or call-center conversations between customers and agents you run into the problem that it becomes very expensive to develop altLabels in a taxonomy tool. We created special software to facilitate the automatic creation of altlabels.
NLP: Basic Pattern Matching for relationship and event detection
Getting entities out of text is now well understood and supported by the software community. However, to find complex concepts or relationships between entities or even events is way harder and requires a flexible rule-based pattern matcher. Given our long time background in Lisp and Prolog one can imagine we created a very powerful pattern matcher.
SPARQL Access
Currently all the features above can be controlled as stored procedures or using Lisp as the command language. We have a new (beta) version that uses SPARQL for most of the control. Here are some examples. Note that fai is a magic-property namespace for “AI”-related stuff and inc is a custom namespace of an imaginary client:
1. Entity extraction
select ?ent {
?subj fai:entityTaxonomy inc:products .
?subj fai:entityTaxonomy inc:salesTerms .
?subj fai:textPredicate inc:text .
?subj fai:entity(fai:language "en", fai:taxonomy inc:products) ?ent .
}
The expressions ?subj fai:entityTaxonomy inc:poducts and ?subj fai:entityTaxonomy inc:salesTerms specify which taxonomies to use (the appropriate matchers are cached).
The expression ?subj fai:entity ?ent will either return the already extracted entities with the specified predicate (fai:entity) or extract the new entities according to the taxonomies in the texts accessible by fai:textPredicate.
2. fai:sentiment will return a single triple with sentiment score:
select ?sentiment {
?subj fai:textPredicate inc:text .
?subj fai:sentiment ?sentiment .
?subj fai:language "en" .
?subj fai:sentimentTaxonomy franz:sentiwords .
}
3. Text classification:
Provided inc:customClassifier was already trained previously, this query will return labels for all texts as a result of classification.
select ?label {
?subj fai:textPredicate inc:text .
?subj fai:classifier inc:customClassifier .
?subj fai:classify ?label .
?label fai:storeResultPredicate inc:label .
}
Further Development
Our team is currently working on these new features:
- A more accessible UI (python client & web) to facilitate NLP and ML pipelines
- Addition of various classifier models
- Sequence classification support (already implemented for a customer project)
- Pre-trained models shipped with AllegroGraph (e.g. English NER)
- Graph ML algorithms (deepwalk, Google Expander)
- Clustering algorithms (k-means, OPTICS)