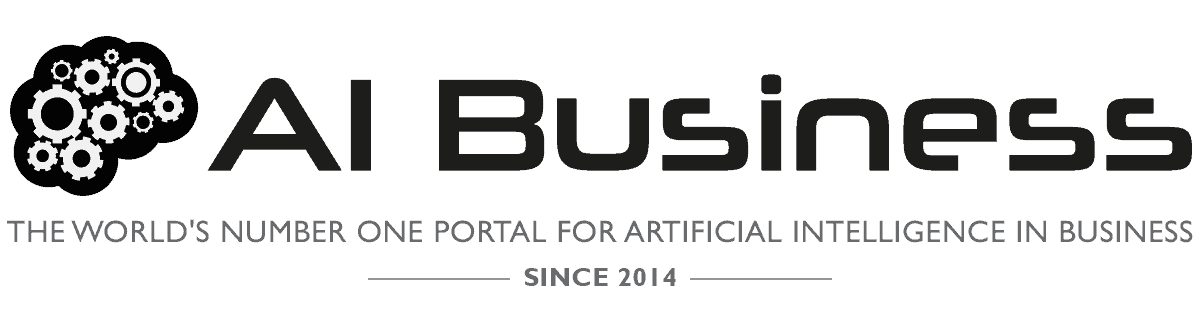
- 20 December, 2018
2019 Trends In The Internet Of Things: The Makings Of An Intelligent IoT
AI Business – December 2018
2019 will be a crucial year for the Internet of Things for two reasons. Firstly, many of the initial predictions for this application of big data prognosticated a future whereby at the start of the next decade there would be billions of connected devices all simultaneously producing sensor data. The IoT is just a year away from making good on those claims.
Dr. Jans Aasman, Franz’s CEO was quoted by the author:
The IIoT is the evolution of the IoT that will give it meaning and help it actualize the number of connected devices forecast for the start of the next decade. The IIoT will encompass smart cities, edge devices, wearables, deep learning and classic machine learning alongside lesser acknowledged elements of AI in a basic paradigm in which, according to Franz CEO Jans Aasman, “you can look at the past and learn from certain situations what’s likely going to happen. You feed it in your [IoT] system and it does better… then you look at what actually happened and it goes back in your machine learning system. That will be your feedback loop.”
Although deep learning relies on many of the same concepts as traditional machine learning, with “deep learning it’s just that you do it with more computers and more intermediate layers,” Aasman said, which results in higher accuracy levels.
The feedback mechanism described by Aasman has such a tremendous capacity to reform data-driven businesses because of the speed of the iterations provided by low latency IIoT data.
One of the critical learning facets the latter produces involves optimization, such as determining the best way to optimize route deliveries encompassing a host of factors based on dedicated rules about them. “There’s no way in [Hades] that a machine learning system would be able to do the complex scheduling of 6,000 people,” Aasman declared. “That’s a really complicated thing where you have to think of every factor for every person.”
However, constraint systems utilizing multi-step reasoning can regularly complete such tasks and the optimization activities for smart cities. Aasman commented that for smart cities, semantic inferencing systems can incorporate data from traffic patterns and stop lights, weather predictions, the time of year, and data about specific businesses and their customers to devise rules for optimal event scheduling. Once the events actually take place, their results—as determined by KPIs—can be analyzed with machine learning to issue future predictions about how to better those results in what Aasman called “a beautiful feedback loop between a machine learning system and a rules-based system.”
In almost all of the examples discussed above, the IIoT incorporates cognitive computing “so humans can take action for better business results,” Aasman acknowledged. The means by which these advantages are created are practically limitless.
Read the Full Article at AI Business.